Analysis of Surface Reaction Mechanism between ALD Precursor and Substrate
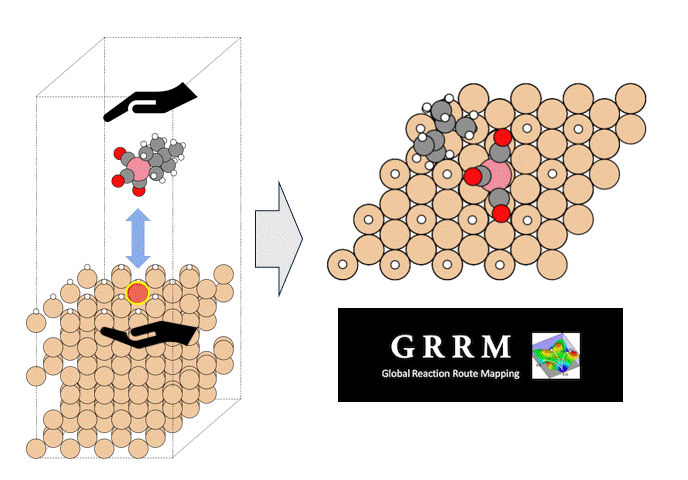
Introduction
Atomic Layer Deposition (ALD) is an important technology for the film deposition process in semiconductor manufacturing, in which volatile organometallic compounds containing the metal to be deposited are used as reaction reagents (precursors). Various combinations of target substrates and metals have been reported depending on the application, but in many cases, the details of their reaction mechanism on the substrate surface are unclear. In order to realize rational precursor design for future applications and development of ALD technology, it is extremely important to understand the surface reaction mechanism at the molecular level. Kwon and Chabai et al. have reported on the reaction of allyl-carbonyl cobalt precursors (1) with hydrogen-terminated Si(111) using DFT calculations.[1] However, in this report, a small cluster structure with only -20 atoms was used as the surface model, and the transition state structures (TS) were not calculated. Here, we performed a reaction analysis using a slab model consisting of -200 atoms in order to elucidate the reaction mechanism of the same reaction. Using the GRRM20 (GRRM20 with Matlantis) program[2, 3], the minimum energy pathway for this complex surface reaction was successfully determined through an automatic and comprehensive reaction pathway search. Furthermore, the same calculations for another cobalt precursor (2) with different allyl ligands was also performed, and showed that quantitative design of the precursor is possible.
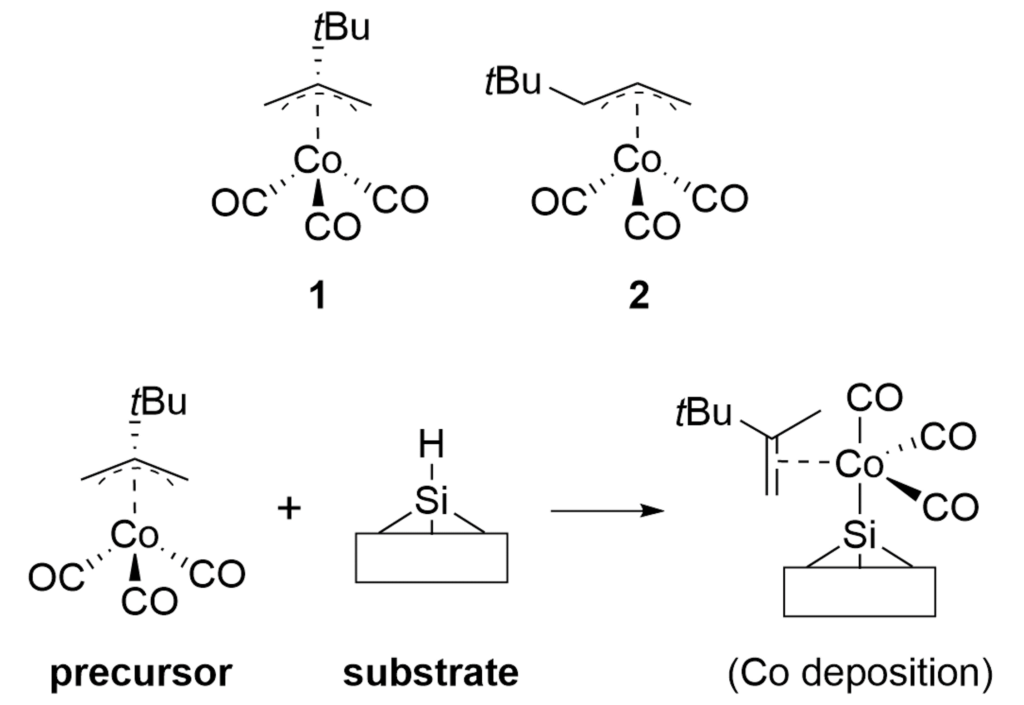
Figure 1. Co precursors and expected surface reaction on H-terminated Si surface.
Computational Details
As a model for a hydrogen-terminated Si surface, the surface structure was cut out from the Si crystal at the 111 plane, and the surface was terminated with hydrogen atoms. Reaction pathway searches were performed for this slab model and precursor using MC-AFIR (Multi Component Artificial Force Induced Reaction).[4] In this process, structural optimization calculations were carried out with artificial forces applied to Co and Si atoms, starting from an initial structure in which the precursor randomly rotated and located on the slab. This process was repeated until no new equilibrium structures (EQ) were found within a specified number of times, thereby achieving an automatic and comprehensive search. After path relaxations of the MC-AFIR pathways using Repath (in GRRM20), 17 and 45 EQs and 12 and 87 TSs were found for 1 and 2, respectively. The energy minimum paths were extracted from the obtained structures, and energy profiles were constructed.
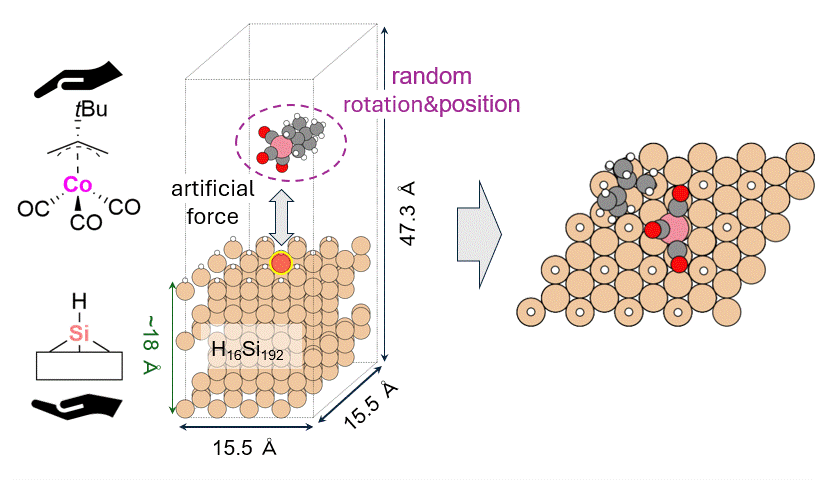
Results and Discussion
By combining Matlantis and MC-AFIR, the reaction pathway search was completed in about one week, a time scale that is inaccessible with conventional DFT calculations. The minimum energy path for both 1 and 2 was found to be a two-step reaction: the insertion of Co into Si-H bond (oxidative addition) and the de-insertion of H and the allyl group (reductive elimination). This reaction mechanism is reminiscent of homogeneous Co-catalyzed organic reactions,[5] and is a reasonable result that is consistent with chemical intuition. In addition, the intermediate of the oxidative addition (INT-OA) took a conformation that was not considered in the reference [1], suggesting that comprehensive searching has eliminated any artificial oversights. From the energy profile of 1, the oxidative addition was the rate-determining step and the activation energy was calculated to be 1.10 eV. This result suggests that the reaction will proceed easily under thermal ALD conditions of around 100°C, and supports the experimental fact that thermal ALD of 1 proceeds smoothly. On the other hand, the corresponding activation energy for 2 decreased significantly to 0.89 eV, indicating that oxidative addition is much faster than for 1. The energy difference is explained by comparing the positions of the tBu groups in the TS (TS-OA) for the oxidative addition. For 1, the tBu group would collide with the surface atoms, whereas for 2, it can be oriented to avoid steric hindrance with CO and the surface, making it energetically favorable. These results indicate that the relationship between substituents on the precursors and their reactivity can be quantitatively evaluated, suggesting that rational design of precursors is possible through the calculation and evaluation of various structures. For example, by changing the structure of the substrate and performing the same calculations, applications to precursor design aimed at area selective deposition would be possible.
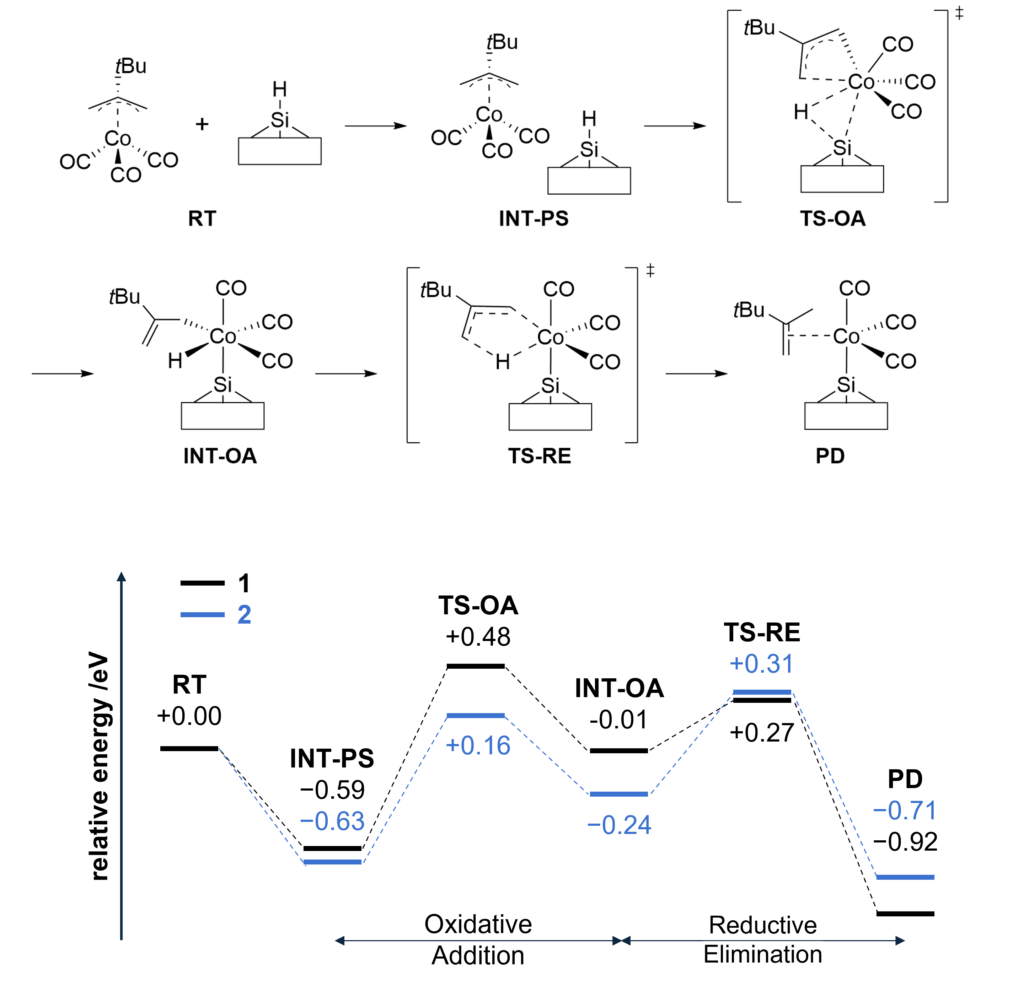
Figure 3. Reaction mechanism of 1 on H-terminated Si(111) and the energy profile of 1 and 2 on H-terminated Si(111).
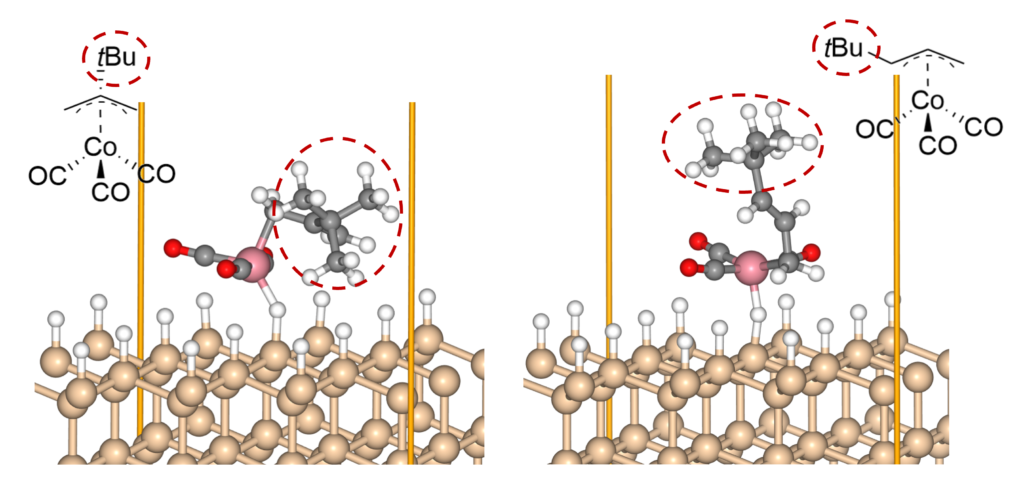
Figure 4. TS-OA for the reaction of 1 and 2 on H-terminated Si(111).
Calculation Conditions
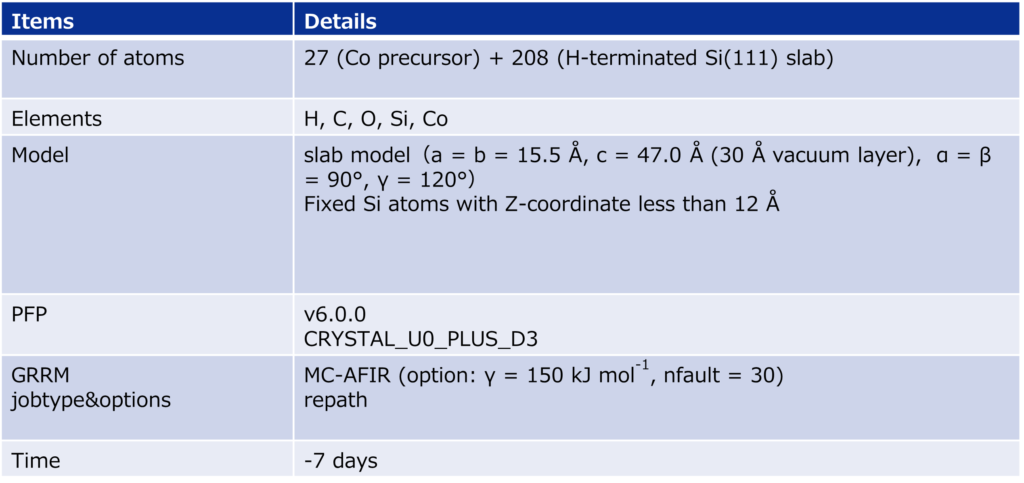