User Testimonials
Simulation Case Studies
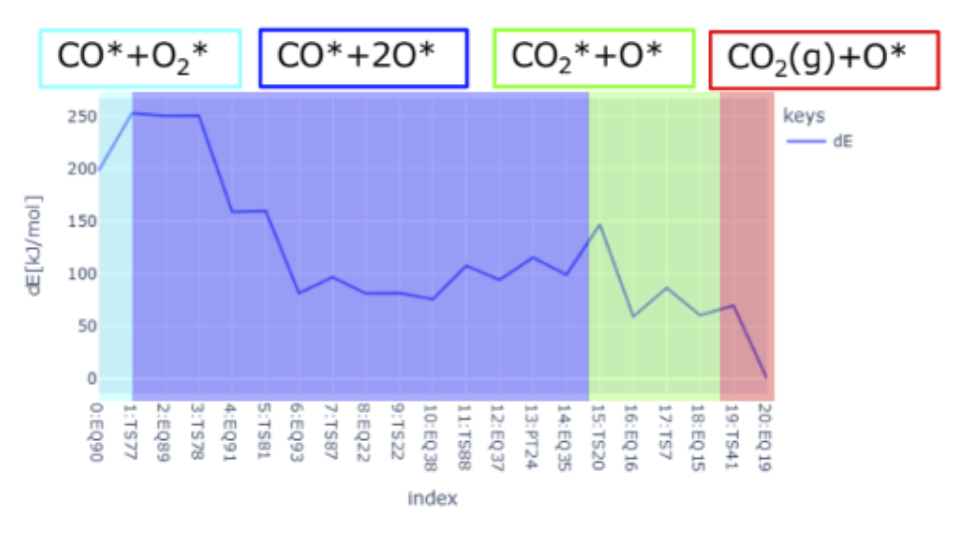
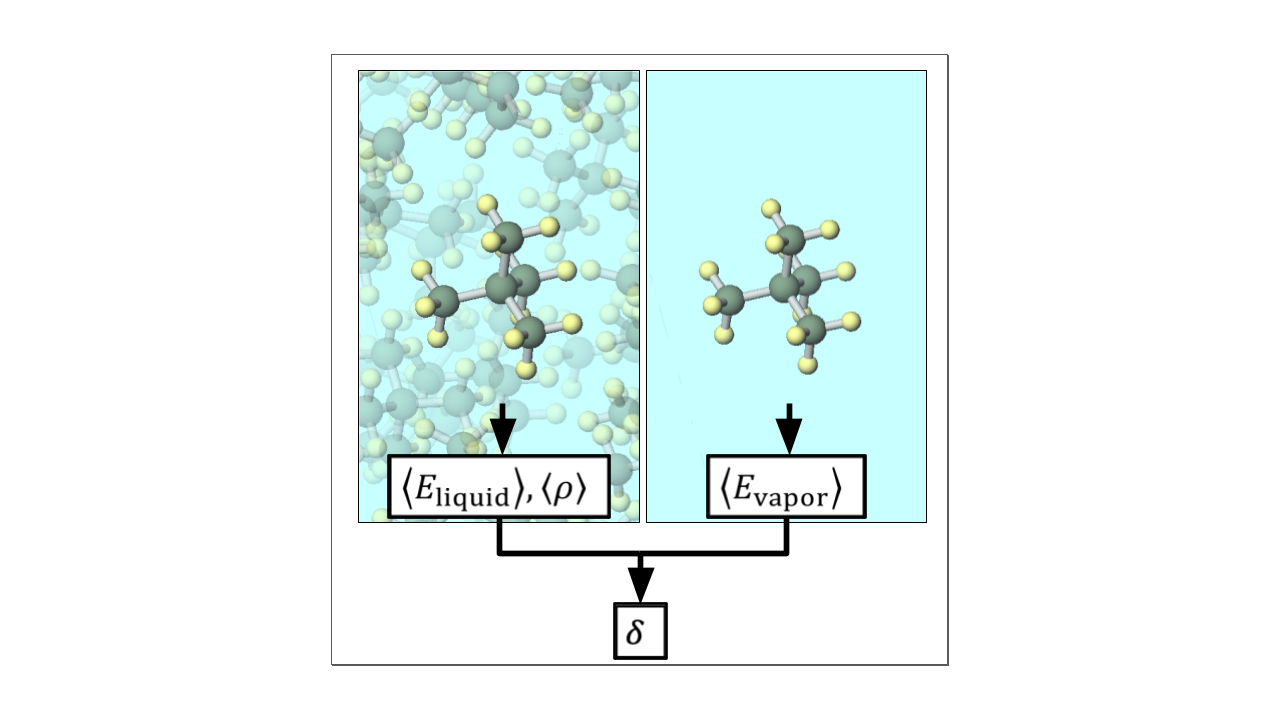
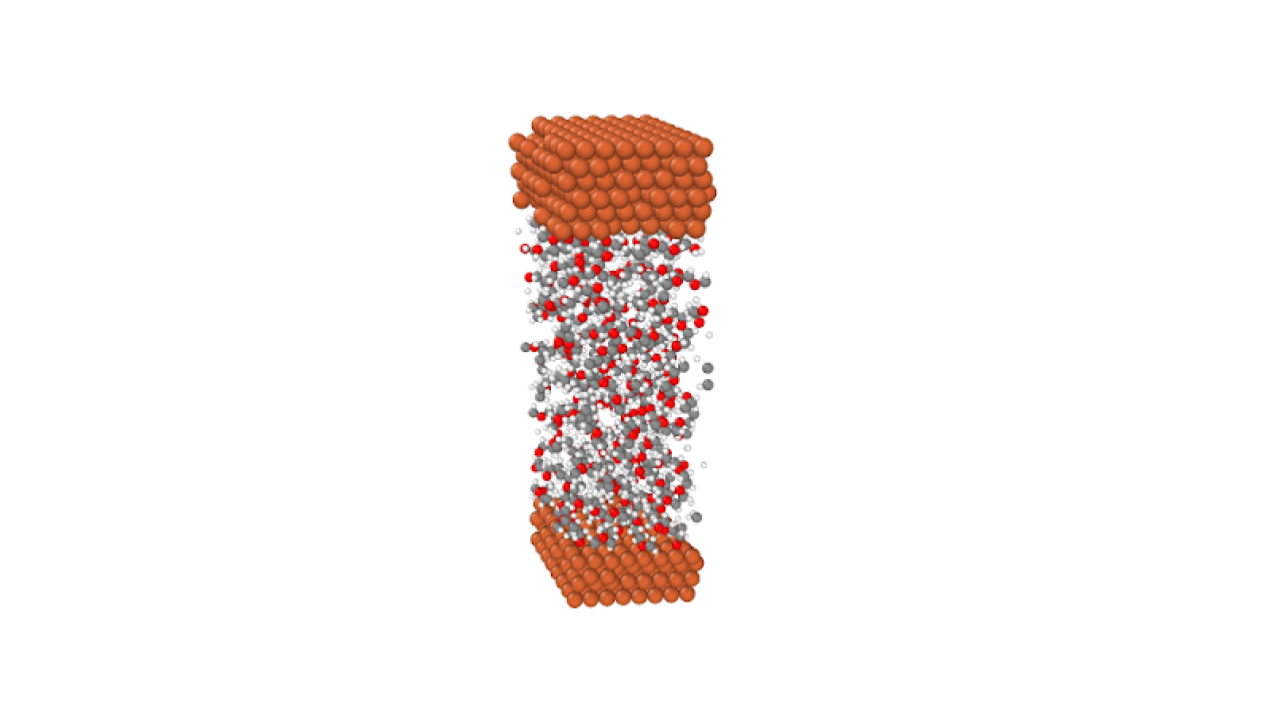
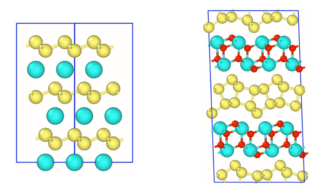
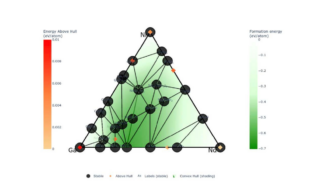
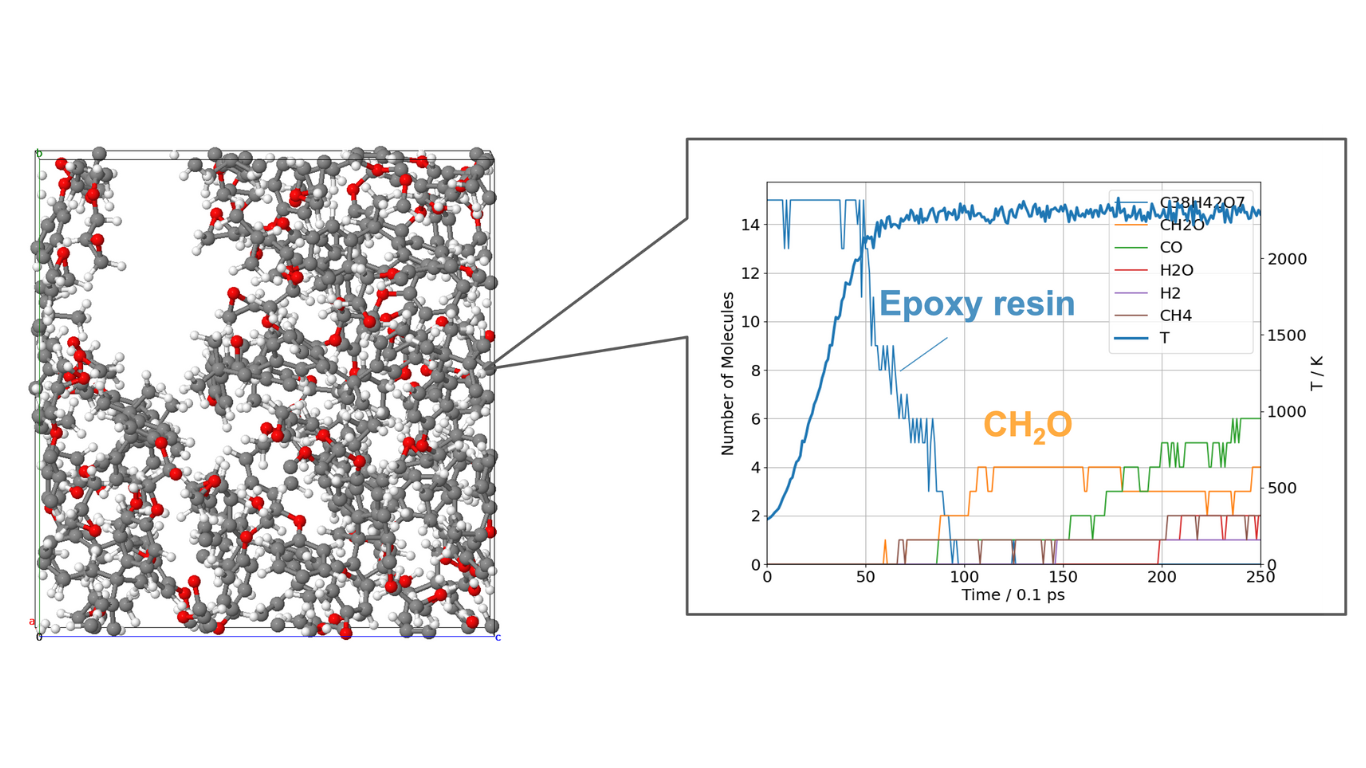
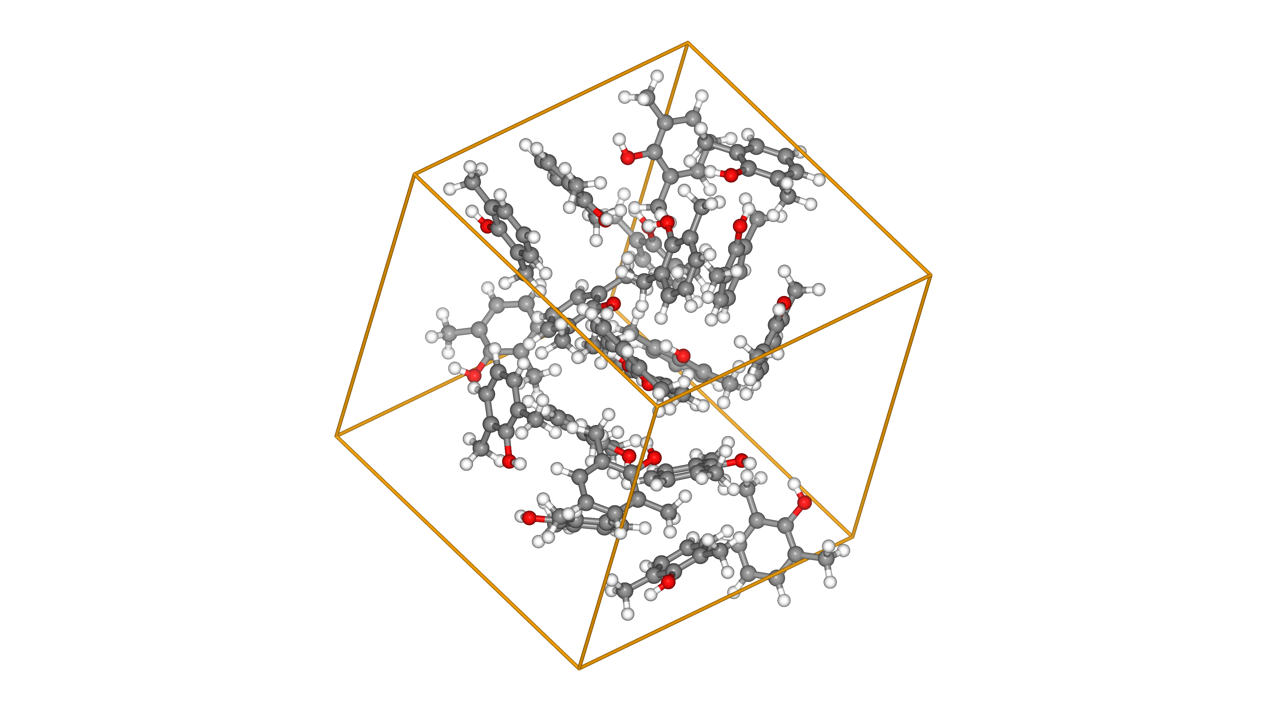
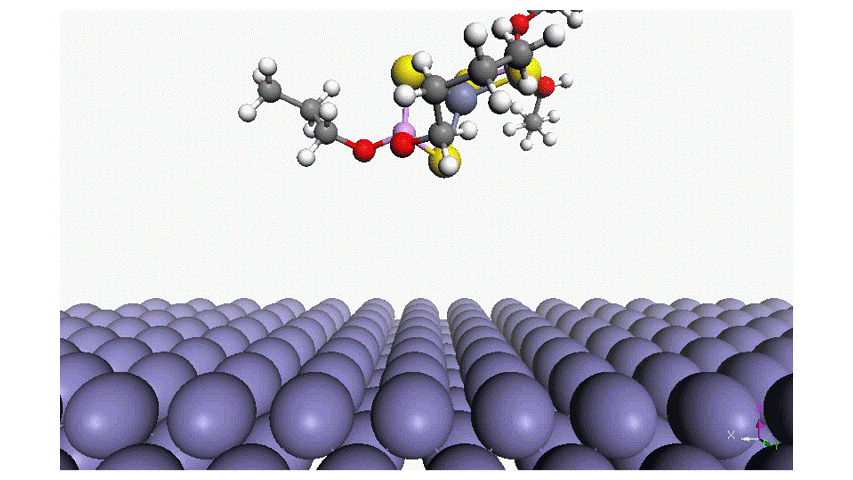
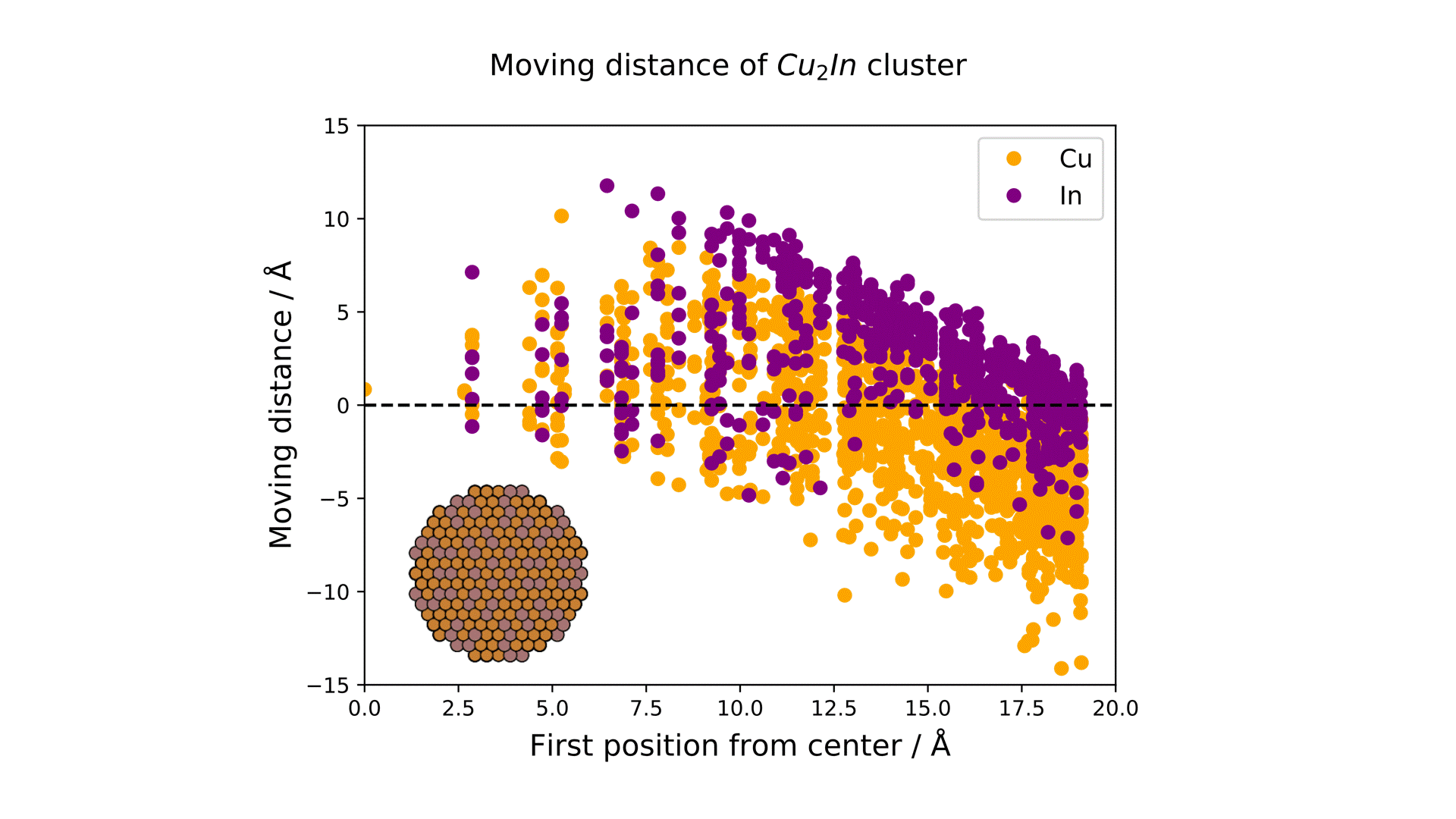
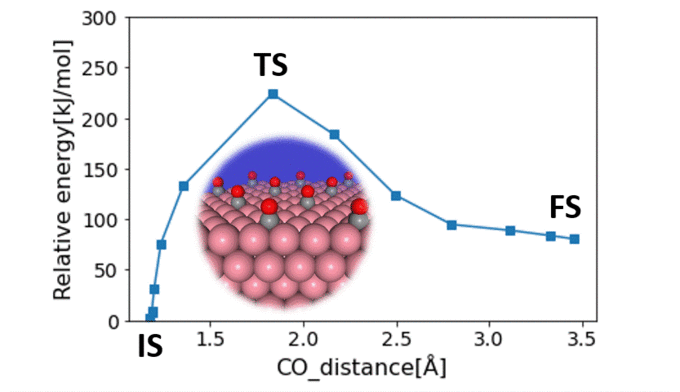
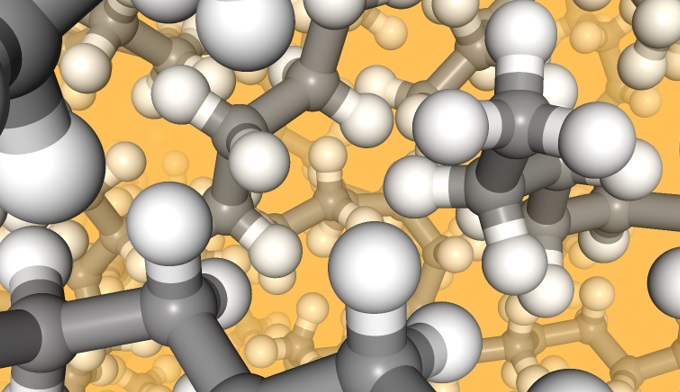
Research Using Matlantis
Quantitative analysis of plasma-enhanced chemical vapor deposition mechanisms: Quantum chemical and plasma-fluid dynamics investigation on tetraethoxysilane/O2 plasma
Hu Li, Kazuki DenpohThis study aimed to investigate the influence of reactive oxygen species (i.e., neutral O atom and O2+ ion) on deposition rates and film thickness uniformity in tetraethoxysilane (TEOS) plasma, utilizing a combination of plasma-fluid dynamic and quantum chemical (QC) simulations. The plasma simulations employed an improved model based on a previous study [H. Li et al., Jpn. J. Appl. Phys. 58, SEED06 (2019)], specifically tailored for a TEOS/O2/Ar/He gas mixture. In the QC simulations, both flat and step silicon oxide (SiO2) surfaces were employed to investigate the adsorption behavior of SiO molecules, the predominant silicon-containing species in TEOS plasma. These simulations also enabled the examination of the rates of SiO molecule adsorption on SiO2 surfaces, facilitating a direct comparison with the sticking coefficients utilized in the plasma simulation. The results of QC simulations revealed that SiO molecules exhibited a higher energetic preference for adsorption on step surfaces than on flat surfaces, resulting in the formation of new SiOH surface sites. Meanwhile, the plasma simulations demonstrated a strong correlation between the deposition rate and film thickness uniformity and the generation of oxygen species, specifically O atoms and O2+ ions, as well as their respective fluxes. This relationship takes precedence over the influence of TEOS or its fragments colliding with the surface. Notably, higher plasma source frequencies were found to enhance the production of atomic O, which contributed significantly to achieving higher deposition rates.
Read moreAdsorption of dimethylaluminum isopropoxide (DMAI) on the Al2O3 surface: A machine-learning potential study
Miso Kim, Sehee Kim, Bonggeun ShongDimethylaluminum isopropoxide (DMAI) is attracting attention as an alternative precursor for atomic layer deposition (ALD) of aluminum oxide (Al2O3). However, the surface chemical reaction mechanisms of DMAI during ALD regarding its dimeric structure under vacuum deposition process conditions has yet to be clear. In this work, the adsorption mechanism of dimeric and monomeric DMAI on a fully hydroxylated Al2O3 surface is studied using machine-learning potential (MLP) calculations. The initial adsorption of DMAI appears facile and would result in the coexistence of both methyl and isopropoxy ligands on the surface. The reactivity of DMAI is smaller than that of TMA, owing to the propensity of DMAI to adopt a dimeric form. Especially when the substrate is partially covered by other adsorbate species, the large molecular size and low reactivity of dimeric DMAI considerably hinder its reactivity toward surface adsorption. Current results are in good correspondence with the previous experimental results, where lower growth per cycle (GPC) and higher selectivity in area-selective ALD (AS-ALD) could be observed by using DMAI than compared to those of TMA processes.
Read moreHigh-throughput investigation of stability and Li diffusion of doped solid electrolytes via neural network potential without configurational knowledge
Ryohto Sawada, Kosuke Nakago, Chikashi Shinagawa & So TakamotoSolid electrolytes hold substantial promise as vital components of all-solid-state batteries. Enhancing their performance necessitates simultaneous improvements in their stability and lithium conductivity. These properties can be calculated using first-principles simulations, provided that the crystal structure of the material and the diffusion pathway through the material are known. However, solid electrolytes typically incorporate dopants to enhance their properties, necessitating the optimization of the dopant configuration for the simulations. Yet, performing such calculations via the first-principles approach is so costly that existing approaches usually rely on predetermined dopant configurations informed by existing knowledge or are limited to systems doped with only a few atoms. The proposed method enables the optimization of the dopant configuration with the support of neural network potential (NNP). Our approach entails the use of molecular dynamics to analyze the diffusion after the optimization of the dopant configuration. The application of our approach to Li10MP2S12-xOx (M = Ge, Si, or Sn) reproduce the experimental results well. Furthermore, analysis of the lithium diffusion pathways suggests that the activation energy of diffusion undergoes a percolation transition. This study demonstrates the effectiveness of NNPs in the systematic exploration of solid electrolytes.
Read moreThe Role of External Donors in Ziegler−Natta Catalysts through Nudged Elastic Band Simulations on Realistic-Scale Models Employing a Universal Neural Network Potential
Masaki Fushimi and Devaiah DammaThis study undertakes a thorough computational exploration of Ziegler–Natta catalysis, emphasizing the role of external donors, particularly dicyclopentyldimethoxysilane (D donor), in the production of polypropylene. Employing the PreFerred Potential (PFP) model within the Nudged Elastic Band (NEB) method and Universal Neural Network Potentials (UNNP), we meticulously assessed the structural integrity of MgCl2 crystals, the dynamics of TiCl4 adsorption, and the kinetics of propylene insertion reactions. Our results demonstrated the precision of the PFP model in accurately replicating the crystalline structures and reaction mechanisms inherent in Ziegler–Natta catalyst systems.A pivotal finding from our research is the significant reduction in activation energy for isotactic propylene insertion, attributed to the presence of at least two D donors around a single Ti active site. Additionally, our computational approach, characterized by its speed and efficiency, successfully incorporates realistic catalyst models, encompassing a range of donor compounds, thereby bridging the gap between theoretical predictions and experimental practices. Our study not only corroborated the existing computational models but also provided novel insights into the mechanistic roles of external donors in Ziegler–Natta catalysis. The implications of these findings extend beyond theoretical studies, offering practical applications in the field of catalytic science and propylene polymerization. This research paves the way for future investigations, potentially transforming our understanding and utilization of Ziegler–Natta catalysts in industrial applications.
Read moreA New Zinc Salt Chemistry for Aqueous Zinc-Metal Batteries
Haoran Du, Yanhao Dong, Qing-Jie Li, Ruirui Zhao, Xiaoqun Qi, Wang-Hay Kan, Liumin Suo, Long Qie, Ju Li, Yunhui HuangAqueous zinc-ion batteries (ZIBs) are promising energy storage solutions with low cost and superior safety, but they suffer from chemical and electrochemical degradations closely related to the electrolyte. Here, a new zinc salt design and a drop-in solution for long cycle-life aqueous ZIBs are reported. The salt Zn(BBI)2 with a rationally designed anion group, N-(benzenesulfonyl)benzenesulfonamide (BBI−), has a special amphiphilic molecular structure, which combines the benefits of hydrophilic and hydrophobic groups to properly tune the solubility and interfacial condition. This new zinc salt does not contain fluorine and is synthesized via a high-yield and low-cost method. It is shown that 1 m Zn(BBI)2 aqueous electrolyte with a widened cathodic stability window effectively stabilizes Zn metal/H2O interface, mitigates chemical and electrochemical degradations, and enables both symmetric and full cells using a zinc-metal electrode.
Read moreSpectroscopic and theoretical analyses of the reaction of SrO in molten chloride and fluoride salts
Dokyu Kang, Choah Kwon, Wonseok Yang, Seokjoo Yoon, Yunu Lee, James T.M. Amphlett, Sang-Eun Bae, Sangtae Kim, Sungyeol ChoiIn this paper, the reaction of SrO in molten chloride and fluoride systems is investigated using Raman spectroscopy, combined with density functional theory calculations. The formation of Sr4OCl6 was observed in various salt compositions, including LiCl, LiCl-KCl, and LiCl-LiF, at temperatures ranging from 298 K to 923 K. Thermodynamic calculations estimated that Sr4OCl6 is more stable than SrO and SrCl2, while no stable strontium oxyfluoride compound was found. The results were also supported by X-ray analyses: Extended X-ray absorption fine structure analysis indicated that the local structure of Sr in LiCl-KCl and LiCl-LiF consists of one oxygen and six chlorides, corresponding to a complex with a similar local structure of Sr4OCl6. Additionally, Sr4OCl6 was found in LiCl-LiF, and no strontium compound was observed in LiF-KF using X-ray diffraction. These results provide a comprehensive understanding of the dissolution structures of strontium oxyhalide in molten chloride and fluoride systems.
Read moreProton Conduction over the Zeolite with Surface Water Cluster for the Water Electrolysis at Neutral Condition
Keigo Tashiro, Taisei Saito, Kojiro Goto, Junki Masuda, Takumi Miyakage, Shuhei Shimoda, Takashi Toyao, Nao Tsunoji, Ken-ichi Shimizu, Hiroshige Matsumoto, Shigeo SatokawaElectrolytic hydrogen production from water at neutral pH was achieved by using novel proton (H+) conduction system assisted by zeolite. Experimental and computational insights demonstrated that defective silanol nest generated by dealumination played an important role in the formation of water clusters and the H+ conduction over the zeolites proceeded via exchange of H-bonding between water molecules in the cluster. In addition, a proper balance between hydrophilicity and hydrophobicity is required for effective H+ conduction and silanol nest afford the balance. Furthermore, the contribution of hydrophilicity of the zeolite for the adsorption of water molecules became more drastic at high temperatures. Water electrolysis efficiency was strongly dependent on the H+ conductivity over the beta-type zeolite. The electrolytic cell containing the zeolite has the potential to be applied to the new hydrogen production system.
Read moreExploration of the mechanical properties of carbon-incorporated amorphous silica using a universal neural network potential
Hiroki Sakakima, Keigo Ogawa, Sakurako Miyazaki, Satoshi IzumiC-incorporated amorphous silica (a-SiOC) is expected to be a significant dielectric film for miniaturized semiconductor devices. However, information on the relationship among its composition, atomic structures, and material properties remains insufficient. This study investigated the dependence of the elastic modulus on the C content in a-SiOC, employing a universal neural network interatomic potential to realize a high-accuracy and high-speed simulation of multicomponent systems. The relationship between elastic modulus and atomic network structures was explored by fabricating 480 amorphous structures through the melt-quenching method without predetermined structure assumptions. The bulk modulus increased from 45 to 60 GPa by incorporating 10% C atoms under O-poor conditions and 20% C atoms under O-rich conditions, respectively. This result is attributed to the formation of denser crosslinking atomic network structures. In particular, the C atoms bonded with the Si atoms with higher coordination under O-poor conditions, whereas they tend to bond with O atoms under O-rich conditions, breaking the SiO2 network. Large C clusters precipitated as the C fraction was increased under O-rich conditions. Gas molecules, such as CO and CO2, were also generated. These results are consistent with reported ab initio calculation results of the formation energies of C defects and gas molecules in SiO2. The findings suggest that realizing O-poor conditions during deposition is crucial for fabricating stronger dielectric films. Therefore, this work contributes to understanding the fabrication of stronger dielectric films and elucidating the underlying mechanism of C cluster formation.
Read moreExploration of elastic moduli of molecular crystals via database screening by pretrained neural network potential
Takuya TaniguchiThe elastic properties of molecular crystals are important for pharmaceutical and material applications, but calculating the elastic constant tensor through theoretical computations is computationally expensive. This study evaluated the feasibility of using neural network potentials, which are noted for their lower computational cost compared to theoretical calculations, in predicting the elastic moduli of molecular crystals. The calculated elastic moduli were sufficiently consistent with experimental values, outperforming Hartree–Fock calculations. It was found that this method is precise enough for predicting the Young's modulus in specific directions via nanoindentation measurements, and relationships between the magnitude of the Young's modulus and crystal structures were also discovered. Furthermore, the database screening of elastic moduli of molecular crystals using a pretrained neural network potential suggested crystals with large and small moduli.
Read moreBoron coordination and three-membered ring formation in sodium borate glasses: a machine-learning molecular dynamics study
Takeyuki Kato, Federica Lodesani, Shingo UrataClassical molecular dynamics (CMD) simulations that employ analytical force fields have been commonly utilized to investigate mechanical, chemical, and thermal properties of oxide glasses owing to their superior computational efficiency. Conversely, simple functional forms limit the accuracy in modeling complicated glass structures, specifically, in alkaline borate glasses, which exhibit boron coordination numbers that vary nonlinearly with changes in glass composition and temperature. Machine-learning potentials (MLPs), which are trained using datasets on energy and force evaluated via the density functional theory (DFT), are garnering significant attention as a novel simulation technology for enhancing the accuracy in modeling materials. Therefore, this study applied a universal MLP, PreFerred Potential (PFP) (trade-name: Matlantis), to model sodium borate glasses, and its accuracy was verified in reproducing boron coordination and ring structures by comparing its results to available experimental data. We found that PFP can quantitatively reproduce the boron coordination change with glass composition without any empirical correction, while the boron coordination in the melts at high temperatures is overestimated, even though the qualitative variation was better estimated than CMD simulations. Furthermore, the MLP could generate many 3-rings, unlike the analytical force-field. Accordingly, we demonstrated superior accuracy of the MLP in modeling alkaline borate glasses, while discussing the challenges faced in reproducing the elaborated microstructures in borate glasses.
Read moreEvolutionary search for superconducting phases in the lanthanum-nitrogen-hydrogen system with universal neural network potential
Takahiro Ishikawa, Yuta Tanaka, Shinji TsuneyukiRecently, Grockowiak et al. reported "hot superconductivity" in ternary or multinary compounds based on lanthanum hydride [A. D. Grockowiak et al., Front. Electron. Mater. 2, 837651 (2022)]. In this paper, we explored thermodynamically stable phases and superconducting phases in the lanthanum-nitrogen-hydrogen system (LaxNyH1−x−y, 0≤x≤1, 0≤y≤1) at pressure of 20GP. We rapidly and accurately constructed the formation-enthalpy convex hull using an evolutionary construction scheme based on density functional theory calculations, extracting the candidates for stable and moderately metastable compounds by the universal neural network potential calculations. The convex hull diagram shows that more than fifty compounds emerge as stable and moderately metastable phases in the region of ΔH≤4.4mRy/atom. In particular, the compounds are concentrated on the line of x=0.5 connecting between LaH and LaN. We found that the superconductivity is gradually enhanced due to N doping for LaH and the superconducting critical temperature Tc reaches 8.77K in La2NH with y=0.25. In addition, we predicted that metastable La2NH2 shows the highest Tc value, 14.41K, of all the ternary compounds predicted in this study. These results suggest that it is difficult to obtain the hot superconductivity in the La-H compounds with N at 20GPa.
Read moreIncreasing the Sodium Metal Electrode Compatibility with the Na3PS4 Solid-State Electrolyte through Heteroatom Substitution
Lieven Bekaert, Suzuno Akatsuka, Naoto Tanibata, Frank De Proft, Annick Hubin, Mesfin Haile Mamme, Masanobu NakayamaRechargeable batteries are essential to the global shift towards renewable energy sources and their storage. At present, improvements in their safety and sustainability are of great importance as part of global sustainable development goals. A major contender in this shift are rechargeable solid-state sodium batteries, as a low-cost, safe, and sustainable alternative to conventional lithium-ion batteries. Recently, solid-state electrolytes with a high ionic conductivity and low flammability have been developed. However, these still face challenges with the highly reactive sodium metal electrode. The study of these electrolyte-electrode interfaces is challenging from a computational and experimental point of view, but recent advances in molecular dynamics neural-network potentials are finally enabling access to these environments compared to more computationally expensive conventional ab-initio techniques. In this study, heteroatom-substituted Na3PS3X1 analogues, where X is sulfur, oxygen, selenium, tellurium, nitrogen, chlorine, and fluorine, are investigated using total-trajectory analysis and neural-network molecular dynamics. It was found that inductive electron-withdrawing and electron-donating effects, alongside differences in heteroatom atomic radius, electronegativity, and valency, influenced the electrolyte reactivity. The Na3PS3O1 oxygen analogue was found to have superior chemical stability against the sodium metal electrode, paving the way towards high-performance, long lifetime and reliable rechargeable solid-state sodium batteries.
Read moreCO Adsorption on Ternary Nanoalloys by Universal Neural Network Potential
Ayako TAMURA, Gerardo VALADEZ HUERTA, Yusuke NANBA, Kaoru HISAMA, Michihisa KOYAMAMulti-element alloy nanoparticles have attracted attention for their potentially high catalytic properties. However, a high degree of freedom in configurations of metal atoms within nanoparticle increases the distinct adsorption sites, making it difficult to theoretically analyze its catalytic properties because the first-principles calculation requires a considerable computational cost. In this study, we develop a sequential scheme to calculate hundreds of adsorption sites by employing a pre-trained universal neural network potential named PFP. Our automated scheme is applied to CO single-molecule adsorption of CO onto PtRuIr ternary alloy nanoparticles. The calculation results are first compared with DFT results to confirm the accuracy. Adsorption energies in the alloy systems are widely distributed in comparison with those of the monometal counterparts, indicating that the alloy nanoparticle includes adsorption sites with various catalytic activities.
Read moreUsing GPT-4 in Parameter Selection of Materials Informatics: Improving Predictive Accuracy Amidst Data Scarcity and ‘Ugly Duckling’ Dilemma
Kan Hatakeyama-Sato, Seigo Watanabe, Naoki Yamane, Yasuhiko Igarashi, Kenichi OyaizuMaterials informatics and cheminformatics struggle with data scarcity, hindering the extraction of significant relationships between structures and properties. The "Ugly Duckling" theorem, suggesting the difficulty of data processing without assumptions or prior knowledge, exacerbates this problem. Current methodologies don't entirely bypass this theorem and may lead to decreased accuracy with unfamiliar data. We propose using Open AI GPT-4 language model for explanatory variable selection, leveraging its extensive knowledge and logical reasoning capabilities to embed domain knowledge in tasks predicting structure-property correlations, such as the refractive index of polymers. This can partially overcome challenges posed by the "Ugly Duckling" theorem and limited data availability.
Read moreOn the Thermodynamic Stability of Alloys: Combination of Neural Network Potential and Wang-Landau Sampling
Tien Quang Nguyen, Yusuke Nanba, and Michihisa KoyamaThermodynamic properties and atomic configuration of PdRu alloy were investigated using Wang-Landau Monte Carlo method in combination with a newly-developed universal neural network potential. By using this new potential, excess energy of PdRu alloy was calculated. It is found that PdRu alloy in FCC lattice is unstable in the full range of alloy composition. This agrees with previous study based on density functional theory. The combined method was able to determine the configurational density of states, from which thermodynamic properties of the alloy were derived. It is found that when temperature increases, the excess free energy of the alloy is reduced, increasing the possibility of alloy mixing. Depending on the composition, transition peaks appear at finite temperatures where there are changes of preferable atomic arrangement due to the effect of temperature via the configurational entropy. In addition, the analyses on short-range order parameter and bond fraction show that PdRu alloy prefers to be in segregated form, where Pd and Ru are immiscible at low temperature, consistent with the experimental observations. The random mixing of Pd and Ru atoms in the form of solid-solution can occur at high temperature.
Read moreAssessing the Reactivity of the Na3PS4 Solid-State Electrolyte with the Sodium Metal Negative Electrode Using Total Trajectory Analysis with Neural-Network Potential Molecular Dynamics
Lieven Bekaert, Suzuno Akatsuka, Naoto Tanibata, Frank De Proft, Annick Hubin, Mesfin Haile Mamme, and Masanobu NakayamaRechargeable batteries play a central role in the global shift from fossil fuels to renewable energy. Since the commercial introduction of lithium-ion batteries in the early 1990s, recent progress is focused on the development of solid-state materials and new battery chemistries. Specifically, solid-state sodium ion batteries are an attractive alternative alongside lithium-based rechargeable batteries, with improvements in safety, lifespan, sustainability, and price. Critical to the battery performance are electrode–electrolyte interfaces since undesirable side-reactions often proceed between electrode and electrolyte materials. In addition, atomistic or electronic-level knowledge on the reactions at the interface is limited due to technical difficulties of experimental observation. Computational studies on interfacial reactivity, such as first-principles techniques, have also long been limited by computational limitations. Recent advances using neural-network potential molecular dynamics simulations are allowing significantly larger systems and longer timescales to be simulated at a significantly reduced computational cost without loss of accuracy. In this study, the chemical stability of the glass–ceramic Na3PS4 solid-state electrolyte with the sodium metal electrode is investigated through a combined total trajectory analysis computational and experimental approach. PS4 groups in the Na3PS4 material were found to decompose sequentially into PS3, PS2, PS, and phosphide and sulfide species through the insertion of sodium atoms. Whereas the decomposition is thermodynamically favored, it is kinetically hindered due to steric effects in the PS3 intermediate. Machine learning-assisted analysis was found to be able to visualize the reactivity tendencies of individual element types. The formed SEI layer exhibited a good chemical stability and a low electronic conductivity. These findings provide new design principles to optimize and develop new solid-state electrolytes with an increased chemical stability toward the sodium metal electrode.
Read moreQuantum Annealing Boosts Prediction of Multimolecular Adsorption on Solid Surfaces Avoiding Combinatorial Explosion
Hiroshi Sampei, Koki Saegusa, Kenshin Chishima, Takuma Higo, Shu Tanaka, Yoshihiro Yayama, Makoto Nakamura, Koichi Kimura, and Yasushi SekineQuantum annealing has been used to predict molecular adsorption on solid surfaces. Evaluation of adsorption, which takes place in all solid surface reactions, is a crucially important subject for study in various fields. However, predicting the most stable coordination by theoretical calculations is challenging for multimolecular adsorption because there are numerous candidates. This report presents a novel method for quick adsorption coordination searches using the quantum annealing principle without combinatorial explosion. This method exhibited much faster search and more stable molecular arrangement findings than conventional methods did, particularly in a high coverage region. We were able to complete a configurational prediction of the adsorption of 16 molecules in 2286 s (including 2154 s for preparation, only required once), whereas previously it has taken 38 601 s. This approach accelerates the tuning of adsorption behavior, especially in composite materials and large-scale modeling, which possess more combinations of molecular configurations.
Read moreEffect of HFO Refrigerants on Lubrication Characteristics (Part 2) -Adsorption Characteristics of Various Refrigerants on Nascent Iron Surfaces and Molecular Simulation Analysis-
Yuji Shitara, Tasuku Onodera, Shigeyuki MoriFollowing the previous paper focusing on tribological properties of HFO (hydro fluoro olefin) refrigerant, the adsorption behavior of refrigerants on the nascent iron surface was investigated experimentally and the adsorption structure, adsorption energy and dynamic process of chemical reaction of refrigerants were analyzed by a molecular simulation. The adsorption behavior on the nascent iron surface was highly dependent on the molecular structure of the refrigerant. HFO refrigerants with an unsaturated bond exhibited high adsorption activity, and halogen species also affected the adsorption activity. HFO showed higher adsorption activity than organic ester, phosphate ester and alkyl sulfide as model compounds of refrigerator oil. In adsorption simulation by neural network potential (NNP), HFO molecules showed large negative adsorption energy. To understand the mechanism for this stronger adsorption of HFO species, density functional theory calculation was conducted, and it showed that HFO adsorbs on iron surface by electron donation from the molecule and back-donation from iron surface. There was also a good correlation between the experimental adsorption activity and the NNP-obtained adsorption energy. MD simulation of molecule adsorbed on the nascent surface at temperature of 298 K was subsequently done using NNP technique. The results showed that CF₃CF = CH₂ (R1234yf) exhibits a distinct decomposition reaction with releasing F atoms and it generates several Fe-F bonds, meaning that precursor of iron fluorides forms on the surface. It is worthy to mention that a formation of the iron fluoride has been experimentally detected on friction track by using XPS in the first report of this study. It was concluded that the adsorption and tribochemical formation of iron fluoride from HFO are supported by molecular simulation performed in this study.
Read moreEffect of HFO Refrigerants on Lubrication Characteristics (Part 1) -Tribological Characteristics under Refrigerant Atmosphere and Adsorption Characteristics on Nascent Metal Surface-
Yuji Shitara, Shigeyuki MoriFocusing on HFO (Hydro Fluoro Olefin) refrigerant, which is expected to be applied as a green refrigerant, the effect of R1234yf on the tribological characteristics was compared with HFC (Hydro Fluoro Carbon) refrigerant R32. Tribological tests were carried out with and without a model lubricant containing triethyl phosphate as an EP additive. R1234yf showed a lower friction coefficient and better wear resistance than R32. XPS analysis of lubricated tracks revealed that metal fluoride was formed from R1234yf and R32 and higher amount of metal fluoride was observed on the track formed under R1234yf than that under R32. The frictional characteristics of R1234yf were affected by refrigerant pressure and temperature of specimen. The higher the pressure of refrigerant and the temperature of the specimen, the better the tribological characteristics were observed. In order to clarify adsorption of refrigerant on metal surface, scratching tests were carried out under refrigerant atmosphere using a mass spectrometer. Although R32 did not adsorb, R1234yf adsorbed on nascent steel surface formed by scratching. The adsorption rate of R1234yf increased linearly with scratching speed. Since propylene also adsorbed on nascent steel surface, π-electrons in R1234yf play an important role on the adsorption. It can be concluded that R1234yf adsorbs easily on nascent steel surface resulting in formation of iron fluoride which acts as a boundary lubrication layer.
Read moreInnovation in Molecular Simulation Technologies for Tribology Using Artificial Intelligence
Tasuku OnoderaIn this article, a newly developed universal neural network potential (NNP), enabling accelerated molecular simulations, and its application to tribological phenomena were reported. The NNP is based on a graph neural network theory and an original dataset including a huge number of the first-principles calculation results. Two application examples using this method were introduced. One was exploring geometry for a fundamental molecular adsorption of long-chain fatty acid, which is difficult to deal with the conventional first-principles calculations. This new technique successfully observed that coverage of fatty acids on metal surface largely influence on the adsorption structures, i.e., orientation angle and intermolecular interaction of long-chain alkyl group. The second application was a molecular dynamics simulation for understanding influence of base oil structures on adsorption behavior of an oiliness additive. The adsorption time of an oiliness additive in three types of base oil (normal paraffin, isoparaffin, and naphthene) was measured. It was found that an oiliness additive in isoparaffin phase showed the earliest adsorption time among the tested base oils. This study unveiled the adsorption mechanisms of the oiliness additive in the base oils: an oiliness additive can smoothly pass inside the isoparaffin-derived oil film with sparse structure and easily reaches metal surface. We concluded that a rapid tribo-material discovery, as well as analysis on molecular mechanism of tribological phenomena, is expected to be achieved by adapting universal NNP to molecular simulations.
Read moreComparison of Matlantis and VASP bulk formation and surface energies in metal hydrides, carbides, nitrides, oxides, and sulfides
Shinya Mine, Takashi Toyao, Ken-ichi Shimizu, and Yoyo HinumaGeneric neural network potentials without forcing users to train potentials could result in significantly acceleration of total energy calculations. Takamoto et al. [Nat. Commun. (2022), 13, 2991] developed such a deep neural network potential (NNP) and made it available in their Matlantis package. We compared the Matlantis bulk formation, surface, and surface O vacancy formation energies of metal hydrides, carbides, nitrides, oxides, and sulfides with our previously calculated VASP values obtained from first-principles with the PBEsol(+U) functional. Matlantis bulk formation energies were consistently ~0.1 eV/atom larger and the surface energies were typically ~10 meV/Å2 smaller than the VASP counterpart. Surface O vacancy formation energies were generally underestimated within ~0.8 eV. These results suggest that Matlantis energies could serve as a relatively good descriptor of the VASP bulk formation and surface energies.
Read moreMolecular dynamics of electric-field driven ionic systems using a universal neural-network potential
Kaoru Hisama, Gerardo Valadez Huerta, Michihisa KoyamaIonic transport under an electric field bias is the fundamental component of electrochemical devices and processes. A universal neural network potential with Bader charge prediction is integrated into a Langevin thermostat NVT simulation to realize a direct simulation to examine those ion dynamics under an external electric field that is scalable and adaptable for various systems. We calculated the ion conductivity of O2− ions in yttria-stabilized zirconia (YSZ) and protons in hydrochloric acid water solution. The conductivity of YSZ shows a tendency consistent with the one using a Buckingham potential tuned for the system. For HClaq, the proton hopping contributes to the higher conductivity of protons than the counter anion Cl−, suggesting that our method is a promising tool for the ionic system, including chemical reactions and either for solid or liquid.
Read moreCyber Catalysis: N2 Dissociation over Ruthenium Catalyst with Strong Metal-Support Interaction
Gerardo Valadez Huerta, Kaoru Hisama, Katsutoshi Sato, Katsutoshi Nagaoka, Michihisa KoyamaCatalysis informatics is constantly developing, and significant advances in data mining, molecular simulation, and automation for computational design and highthroughput experimentation have been achieved. However, efforts to reveal the mechanisms of complex supported nanoparticle catalysts in cyberspace have proven to be unsuccessful thus far. This study fills this gap by exploring N2 dissociation on a supported Ru nanoparticle as an example using a universal neural network potential. We calculated 200 catalyst configurations consideringthe reduction of the support and strong metal-support interaction (SMSI), eventually performing 15,600 calculations for various N2 adsorption states. After successfully validating our results with experimental IR spectral data, we clarified key N2 dissociation pathways behind the high activity of the SMSI surface and disclosed the maximum activity of catalysts reduced at 650 °C. Our method is well applicable to other complex systems, and we believe it represents a key first step toward the digital transformation of investigations on heterogeneous catalysis.
Read moreCalculations of Real-System Nanoparticles Using Universal Neural Network Potential PFP
Gerardo Valadez Huerta, Yusuke Nanba, Iori Kurata, Kosuke Nakago, So Takamoto, Chikashi Shinagawa, Michihisa KoyamaIt is essential to explore the stability and activity of real-system nanoparticles theoretically. While applications of theoretical methods for this purpose can be found in literature, the expensive computational costs of conventional theoretical methods hinder their massive applications to practical materials design. With the recent development of neural network algorithms along with the advancement of computer systems, neural network potentials have emerged as a promising candidate for the description of a wide range of materials, including metals and molecules, with a reasonable computational time. In this study, we successfully validate a universal neural network potential, PFP, for the description of monometallic Ru nanoparticles, PdRuCu ternary alloy nanoparticles, and the NO adsorption on Rh nanoparticles against first-principles calculations. We further conduct molecular dynamics simulations on the NO-Rh system and challenge the PFP to describe a large, supported Pt nanoparticle system.
Read moreFollow us
Moves to the selected area