ACS FALL 2024出展のお知らせ
PFCCは 2024年8月18日(日)~ 8月22日(木) に開催される「ACS FALL 2024」に出展します。出展内容は、以下ご参照ください。
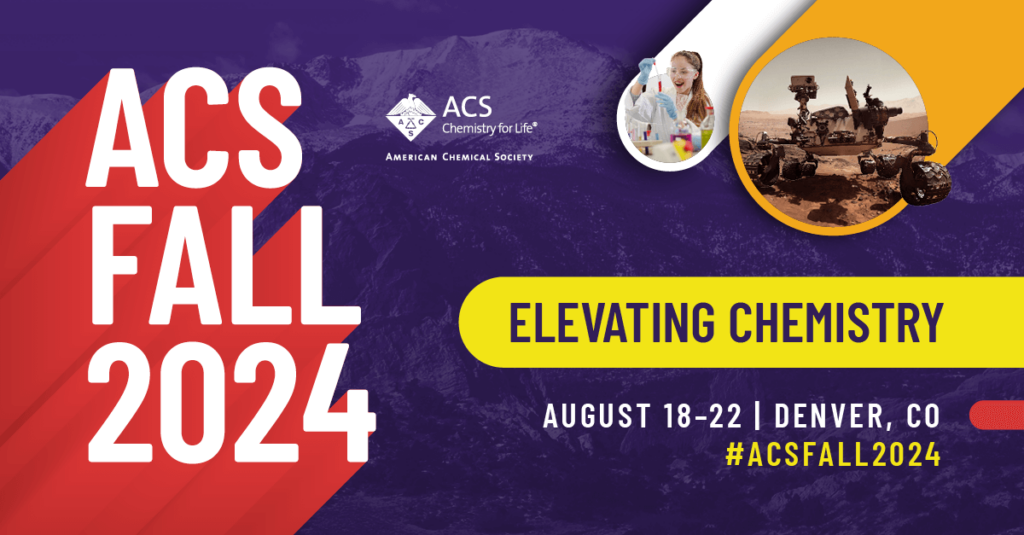
展示会&ブース概要
展示期間 : 2024年8月19日(月)~ 8月21日(水)
開催場所 : Colorado Convention Center, Denver, CO
ブース : 1815
プレゼン情報
DIVISION : Division of Computers in Chemistry
SESSION : Material Science
SESSION TIME : 8:00 AM – 11:45 AM (Local time)
講演日時 : 2024年8月20日(火) 9:30 AM – 9:45 AM
講演会場 : Hall D – Room 1
タイトル : Evaluation of a universal neural network potential for applications to non-ideal structures.
概要 :
Machine learning potentials are promising techniques for accelerating atomistic simulations for materials development. The Preferred Potential (PFP), integrated into Matlantis™, is a recently developed universal graph neural network (GNN) potential trained on large DFT datasets, which include crystals, molecules surfaces, adsorbates and disordered structures1. Such comprehensive training enables PFP to be applied to practical applications in diverse areas, including thermodynamic analysis, reaction kinetics, and molecular dynamics simulations. PFP was shown to perform well in predicting energies and forces reproducing results from the PBE functional, which is used as training data, as well as comparison with experiments1. To ensure the reliable application of universal machine learning potentials, it is crucial to validate their accuracy on specific systems and properties, especially those involving structural imperfections and finite temperatures.
In this study, we have examined the accuracy of PFP for non-ideal structures and thermodynamic properties. Benchmarking of PFP for the grain boundary energies of elemental metals indicate that PFP well reproduces the PBE results. Surface energies for elemental crystals mostly agree well with those of PBE results, while surface energies of transition metals are underestimated. The lower accuracy of these surfaces possibly stem from insufficient training data or from an inaccurate surface magnetism. Specific heat of crystals and organic liquids at finite temperature were modeled using quasi-harmonic phonons and two thermodynamic phase models2, and the PFP results agree well with experiments. These results demonstrate that GNN potentials trained on large dataset well reproduce the potential energy curve around the local minimum with PBE accuracy.
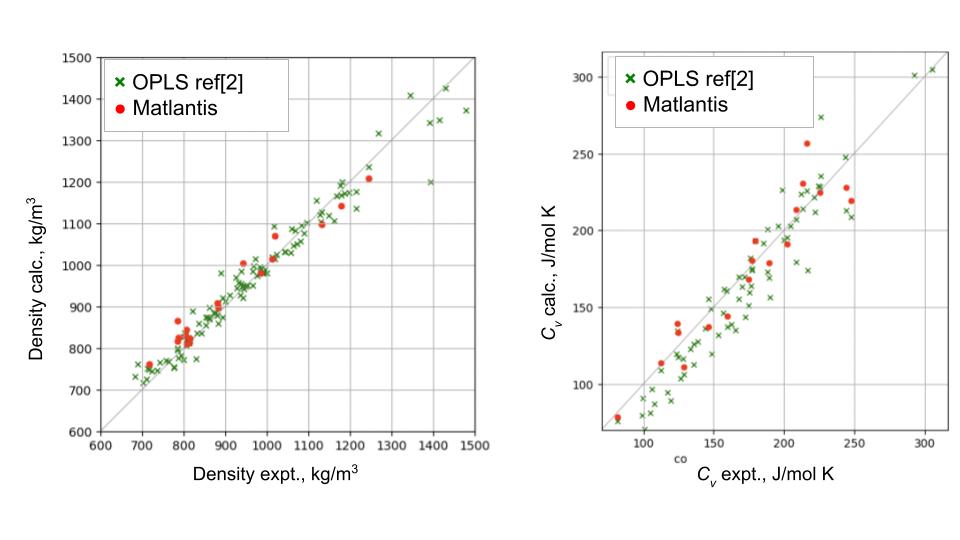
(1) S. Takamoto, et al. Nature Communications 2022, 13, 2991.
(2) C. Caleman, et al. J. Chem. Theory Comput. 2012, 8, 61.