Catalyst Screening for Ammonia Synthesis Catalyst
Case Study Provider:
ENEOS Corporation
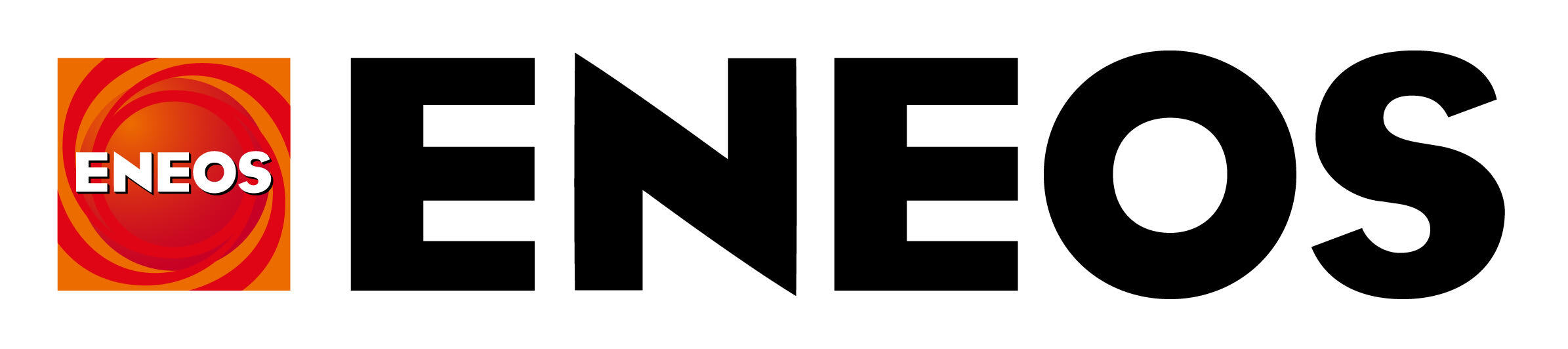
Overview
Heterogeneous catalysts play a crucial role in the production and utilization of fuels, chemicals and pollution remediation. Virtual screening is gaining attention as a method to find new candidate catalysts. One of the most successful methods for catalyst screening is descriptor-based microkinetics, using models based on scaling relationships [1]. In this method, catalytic activity is plotted as a function of one or two descriptor energies, resulting in a volcano-shaped plot. Catalyst designs can be obtained for the optimal catalytic activity or selectivity using this plot.
In this study, fast catalyst screening was performed using Matlantis to calculate descriptor energies. Ammonia synthesis, one of the most important industrial reactions, was selected as the model catalytic reaction. Using Matlantis, the reproduction of DFT calculation results, as well as the virtual screening of new candidate catalysts, were examined.
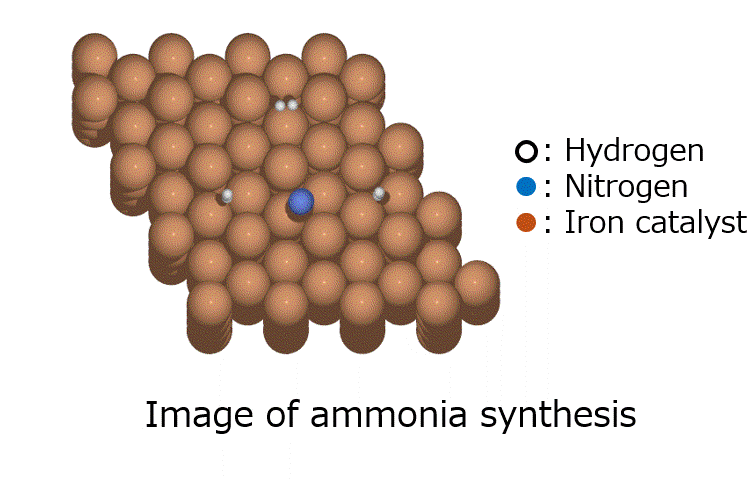
Calculation models and methods
Validation of energy accuracy
Energy calculations were performed using Matlantis for intermediates (slab, H*, N2*, N*, NH*, NH2*, NH3*) and transition states(H-H*, N-N*, N-H*, NH-H*, NH2-H*) related to ammonia synthesis adsorbed on various transition metals. A comparison was made between the PFP and DFT results[2].
(The asterisk (*) symbol indicates adsorbed molecules; for example, N* represents a nitrogen atom adsorbed on the catalyst surface.)
Descriptor-based microkinetics for ammonia synthesis
Using the energies calculated by Matlantis, descriptor-based microkinetic modeling was performed to simulate the ammonia synthesis reaction rate. The calculation tool CatMAP [3] was used. The ammonia synthesis rate was calculated under the conditions of 673K, 100 bar total pressure, H2: N2 = 3:1, and 2% NH3 conversion.
Catalyst screening for ammonia synthesis
Calculations of the N* adsorption energy(EN*) and N-N* transition state energy(EN-N*) were performed on approximately 2,000 different binary alloy catalysts [4]. Finally, the calculated energy results were plotted on a map constructed from the ammonia synthesis rate calculations, enabling virtual screening of new catalysts.
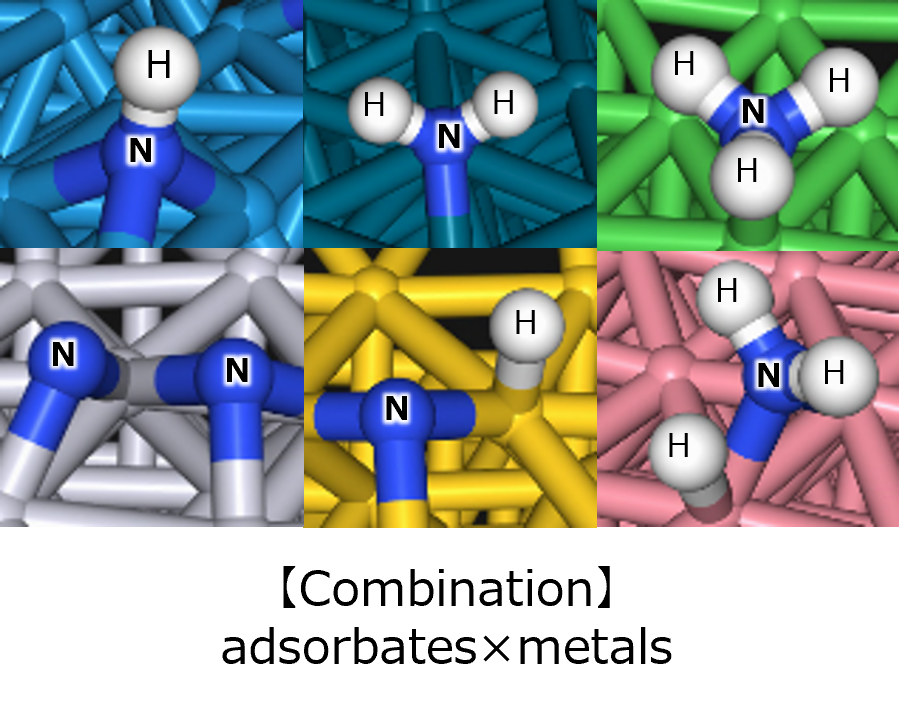
Calculation Results and Discussion
Validation of energy accuracy
This plot shows the comparison between PFP and DFT [2] energies. From the figure, it can be understood that the energy values form a linear pattern, demonstrating that Matlantis can calculate with an accuracy equivalent to DFT(R2=0.94) regardless of the type of metals or adsorbates. This indicates that Matlantis possesses both high universality and accuracy.
Descriptor-based microkinetics for ammonia synthesis
The x axis of the figure represents the adsorption energy of N*(EN*), and the y axis represents the transition state energy of N-N*(EN-N*). The plot shows EN* and EN-N* values for each transition metal calculated by PFP. This study, which combines Matlantis with descriptor-based microkinetics, calculated figures with accuracy comparable to previous DFT work[2].
Catalyst screening for ammonia synthesis
Taking advantage of the high speed, accuracy and universality of Matlantis, EN* and EN-N* calculations were performed on approximately 2,000 different binary alloy catalysts, enabling the virtual screening of new catalysts. As a result, several promising candidate catalysts (red plots) were identified, which are predicted to have high activity for ammonia synthesis.
Although catalyst screening using descriptor-based microkinetics has been constructed in previous studies, it is usually time-consuming, especially when transition states are calculated to determine required descriptors. In this study, multiple promising candidate catalysts were identified in a short period. This method is expected to become a powerful tool for the exploration of new catalysts in the future.
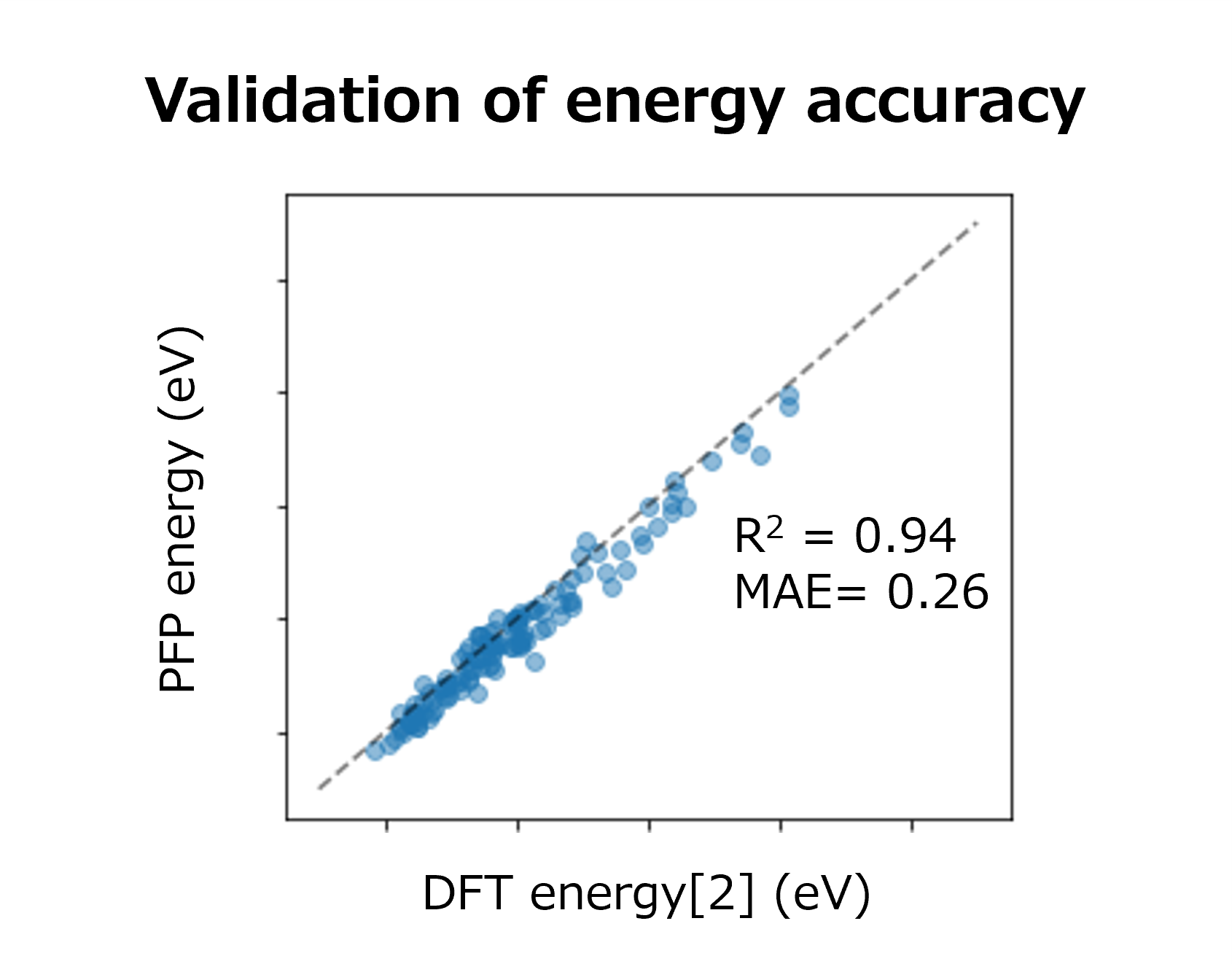
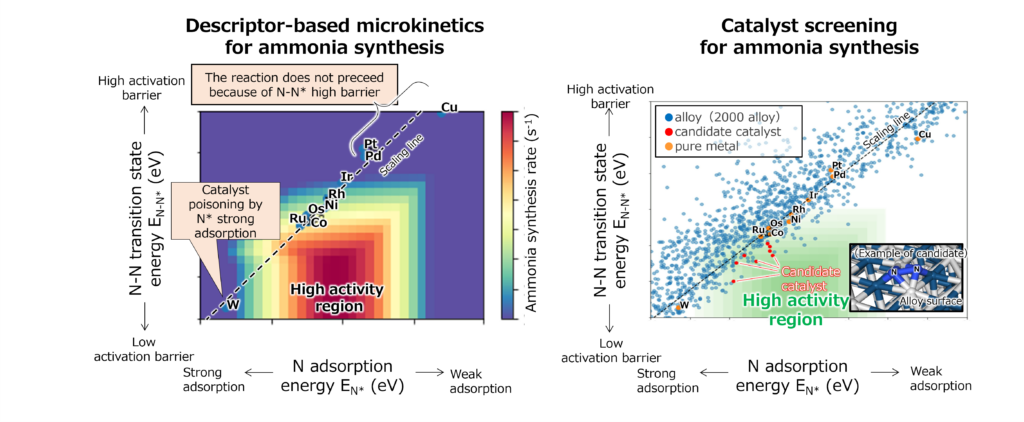
Calculation Conditions
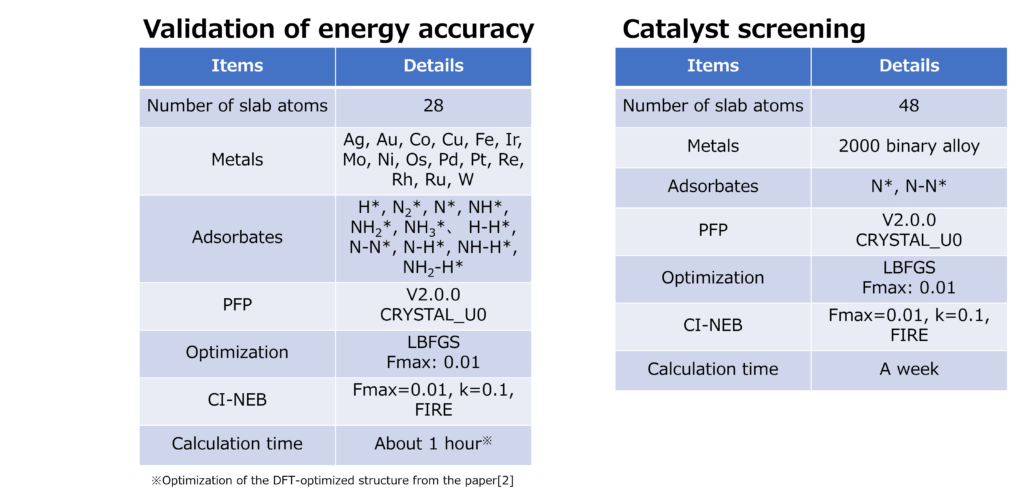