Evaluation of Lithium Ion Conductivity of Garnet Type Oxide Materials
Nagoya Institute of Technology Nakayama lab.
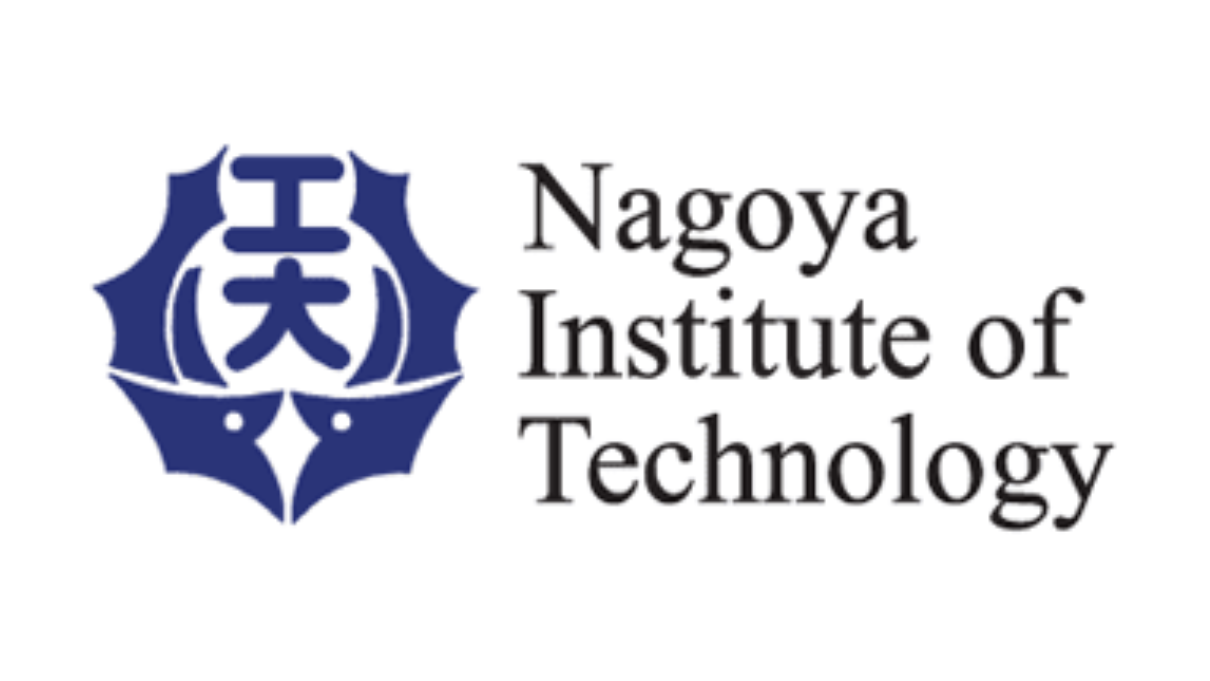
Overview
The risk of ignition and explosion is an issure for current lithium-ion battary, since it uses flammable organic electrolytes. Using non-flammable lithium-ion conductive oxides as solid electrolytes, safe batteries can be created, but it is necessary to find materials with sufficiently high lithium-ion conductivity. First-principles molecular dynamics (FPMD) is a powerful alternative to trial-and-error experimental methods because of its ability to accurately evaluate ionic conductivity. However, FPMD is expansion. Even with the modern computer, it can take weeks or even months to calculate the ionic conductivity of a solid electrolyte of a given composition under a given condition (temperature, etc).
In this study, we performed molecular dynamics (MD) on a garnet-type Li7La3Zr2O12 material, which is a typical oxide solid electrolyte material, using the fast and accurate Matlantis to evaluate Li ion conductivity. We also compared the results with those of FPMD under the same conditions.
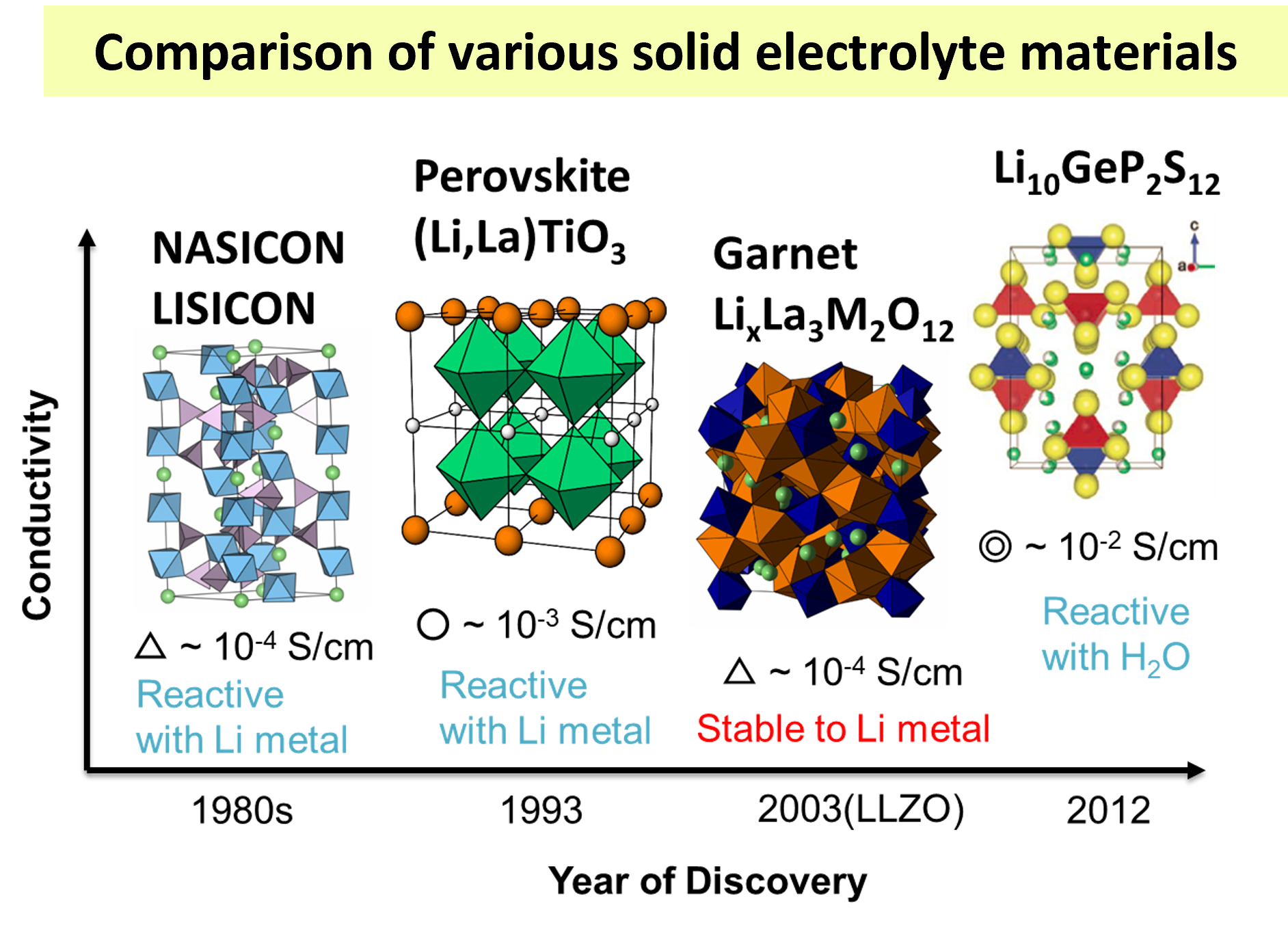
Calculation models and methods
The atomic structure of Li56La26Zr16O96 (contains 192 atoms) was constructed, and it was used as the initial structure for both FPMD and Matlantis-based MD. Both atomic structure and lattice shape is relaxed before the MD run, to determine the appropriate lattice volume. Then, MD simulations were performed under NVT ensemble (constant volume) conditions at a temperature of 1273 K. The FPMD were performed on 32 cores in parallel. The detailed conditions of the FPMD is described in the paper [1].
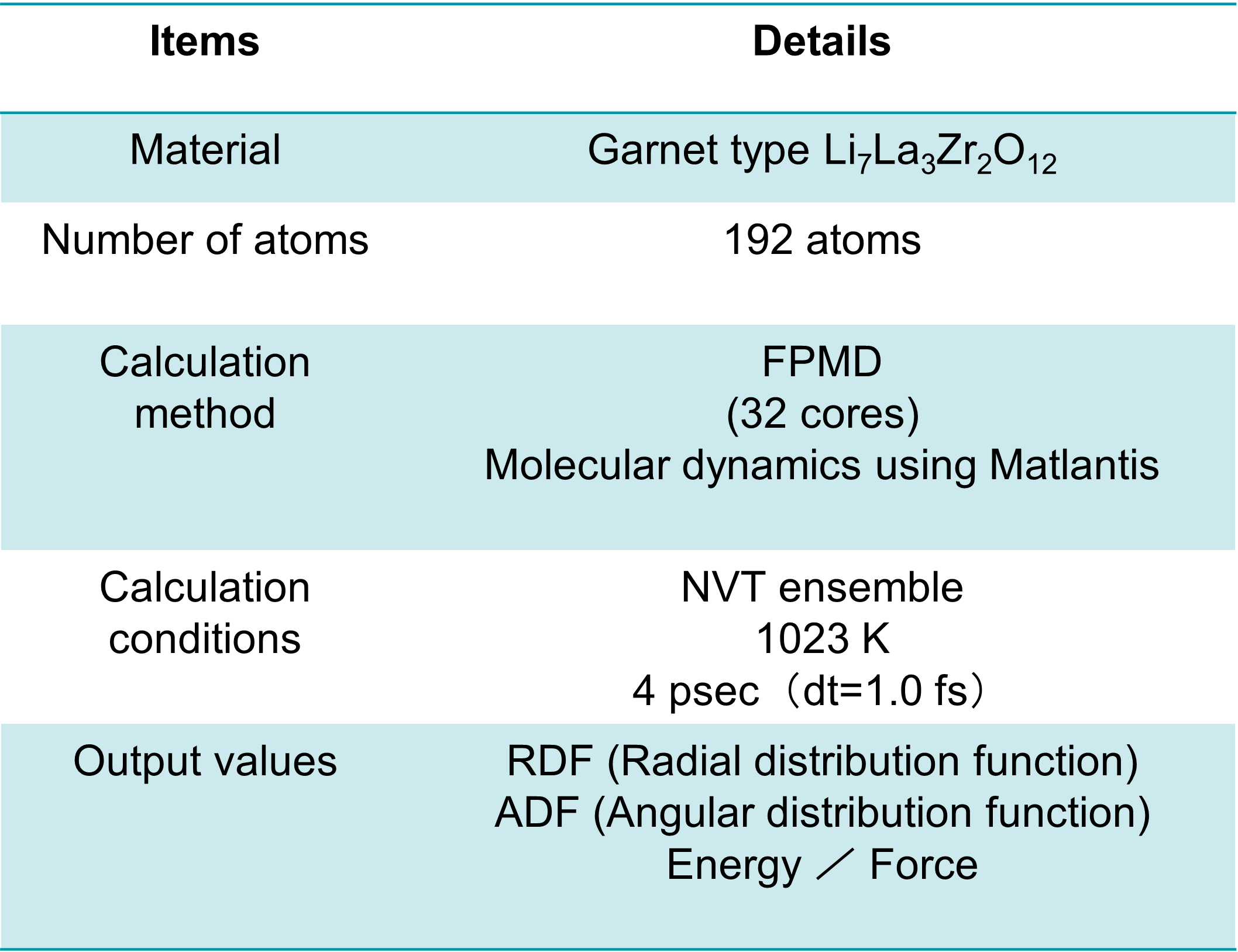
Calculation Results and Discussion
It takes tens of times longer to run the same number of MD steps using FPMD than using Matlantis, which demonstrated the high computation speed of Matlantis. The radial distribution function (RDF), which represents the distribution of distances between atoms, and angular distribution function (ADF), which represents the distribution of bond angles, are used to analyze the MD trajectories, and both of them indicate the very good agreement between FPMD and Matlantis. The snapshots are extracted from the FPMD trajectories, and then the energy and forces acting on each atom was recalculated with Matlantis, so that we can compare the results with first-principles calculations. From the diagnostic plot, we were able to obtain very good agreement between the two.
The above results show that Matlantis makes it possible to perform FPMD calculations an order of magnitude faster. This demonstration was done using a small simulation cell consisting of 192 atoms to compare FPMD and Matlantis, so the difference in calculation speed is moderate. However, Matlantis can perform MD simulations in a reasonable amount of time, even in large simulation cells, which is not possible with FPMD.
It will be possible to evaluate the ionic conductivity of a large amount of garnet-type Li7La3Zr2O12 materials with substitutions in a short time, which is expected to shorten the time required for composition optimization.
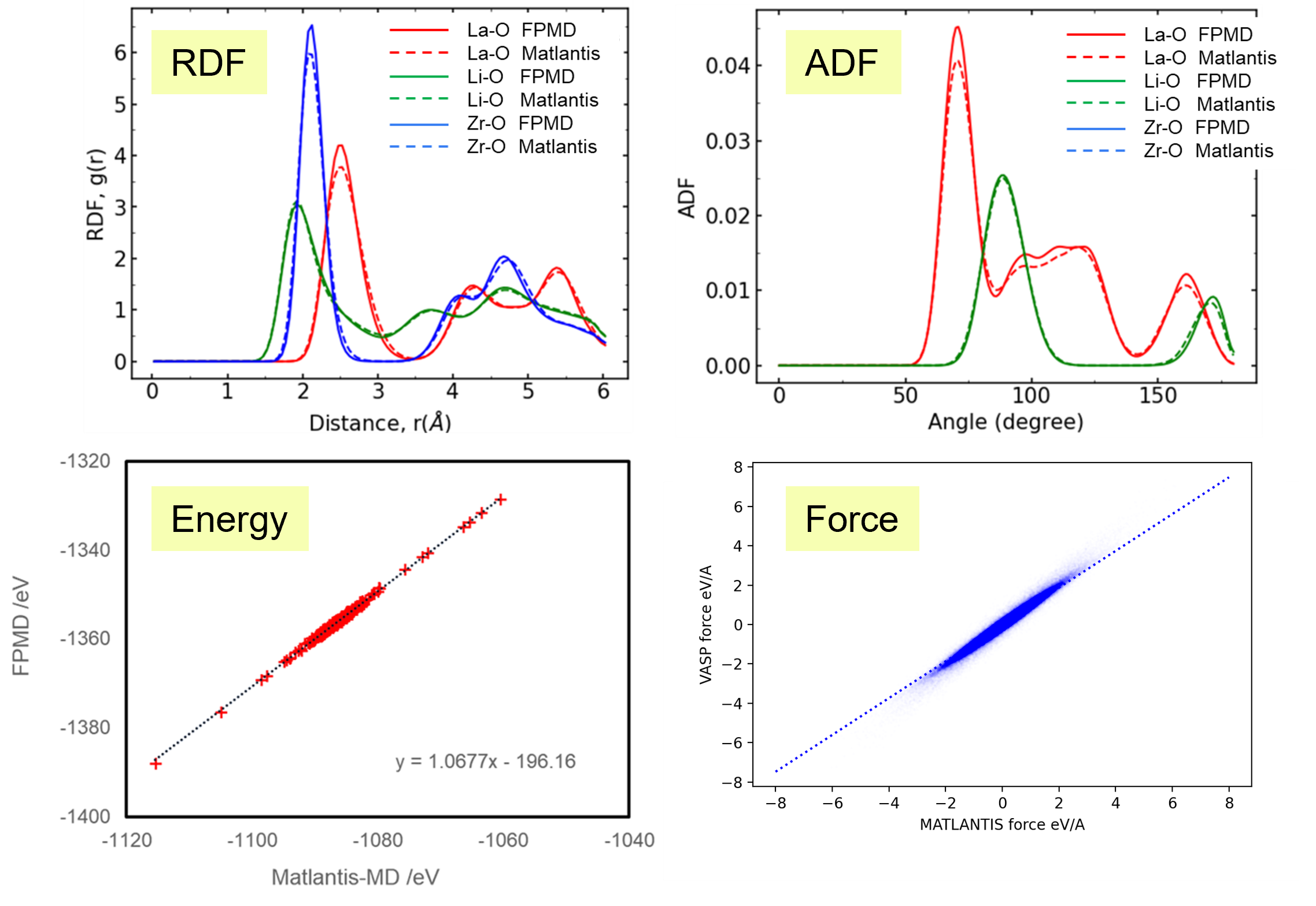