Prediction of fluid viscosity of alkanes by reverse non-equilibrium molecular dynamics simulation
Overview
From the perspective of curbing global warming, there is a growing demand for more energy efficient machinery and equipment such as automobiles and home appliances.
Since one way to save energy is to reduce friction loss of sliding parts, lowering viscosity is strongly necessary for fluids such as lubrication oil as well as lowering friction.
In the design of fluids, it is generally recognized that the method of molecular simulation is effective in predicting the viscosity of mixtures of more than two materials or novel fluids.
As an example, we introduce the result of viscosity calculations for common organic molecules of alkanes using PFP.
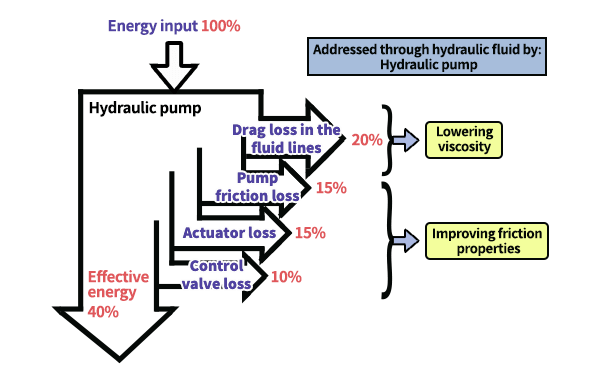
The model and method
As an example of viscosity calculation, pentane, a 5C normal alkane, was selected as a target. Prior to the calculation, a liquid structure of pentane was constructed.
50 molecules of pentane were randomly placed in a simulation cell, and the cell volume was adjusted to maintain the density constant by molecular dynamics MD) method with PFP.
Three simulation models with different initial configurations were prepared to check the reproductivity of the final result.
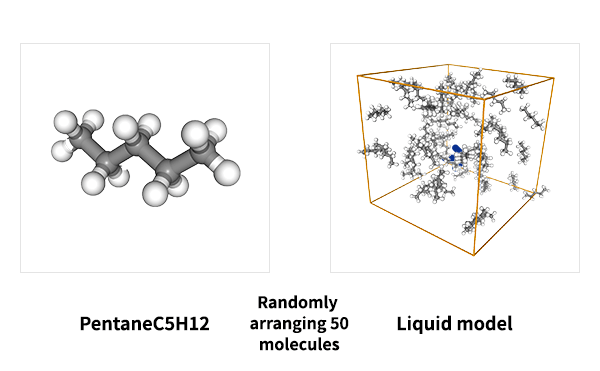
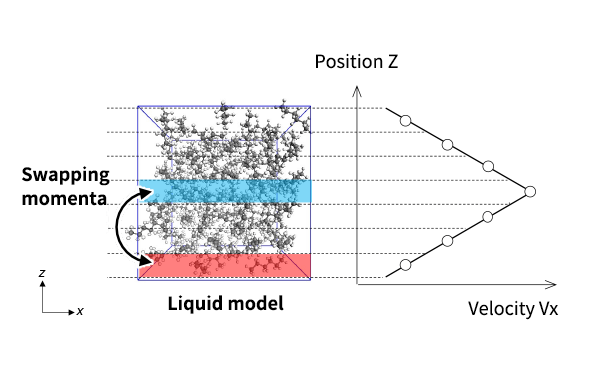
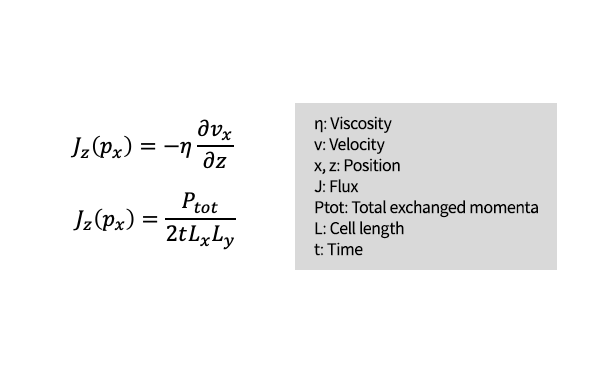
The reverse non-equilibrium molecular dynamics (RNEMD) method [1] was adapted for the purpose of calculating viscosity. This method forcibly induces velocity gradient in a liquid phase by exchanging momenta between atoms that have two opposite velocity signs, positive and negative, in two specific regions
The RNEMD simulations were performed for duration of 2×105 steps for each of the three prepared models with different initial configurations. The integration time and temperature were set to 1 fs and 298 K, respectively.
Results and discussion
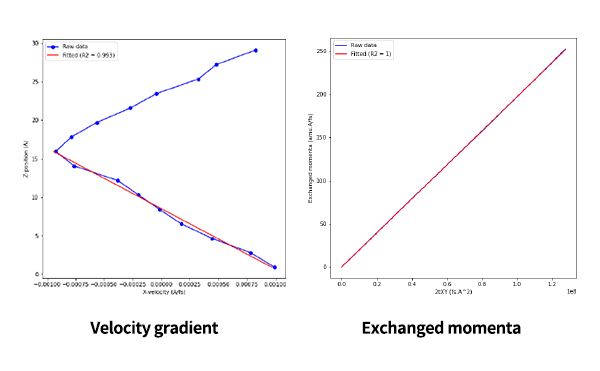
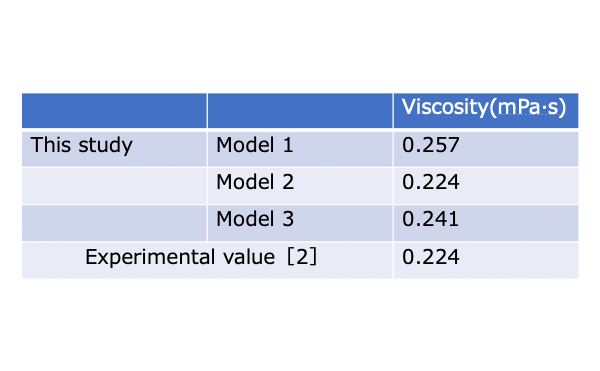
This calculation was done for a simple organic compound, but our PFP possesses remarkable features of high accuracy and universality.
PFP is expected to be applied to physical property prediction of systems where a classical force field is not fixed or needs to be tuned such as ionic liquids and electrolytes.
Calculation Condition
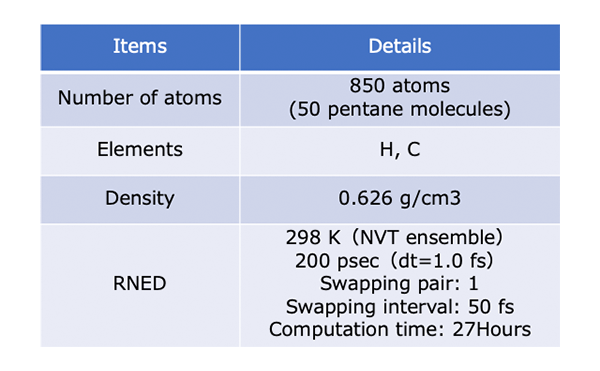