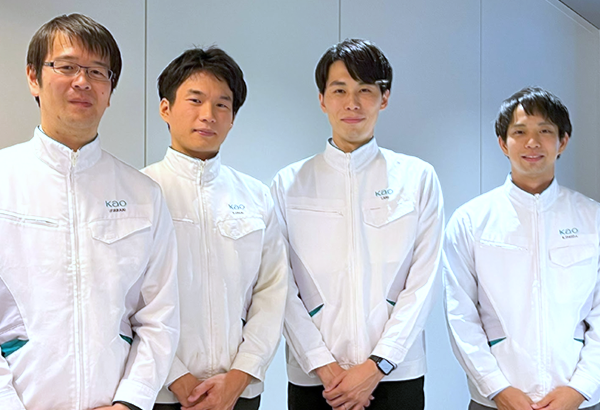
Matlantis User Testimonial
– Kao Corporation
Kao successfully accelerated chemical product development with Matlantis™ which can predict experiment results using computational chemistry. “Our development division expects our computation speed and efficiency to keep pace with their operations. Matlantis quickly paved the way because the calculation speed is tens of thousands of times faster than the previous method.”
Kao Corporation
- Industry
- Chemicals
- Business
- Production and sales of cosmetics, quasi-pharmaceuticals, industrial chemicals, hygiene products, etc.
Kao Corporation has been using materials informatics to shorten product development time, and finding good catalysts has been one of the priorities. Although catalysts are the keys to ESG – environmental, social, and corporate governance – as mentioned in the 12 Principles of Green Chemistry, the number of candidate materials are so huge that conventional technologies require years of development period. Kao has formulated a plan to shorten the period needed for searching the ideal catalysts with the power of computational chemistry. The company successfully shortened the period by adopting Matlantis™. We interviewed four members of Kao’s Analytical Science Laboratory, Research and Development Division about this initiative about the success and their future prospects.
Q. What kind of research and development projects have you been working on?
Kosuke Takeda: In general, the Analytical Science Laboratory primarily uses computational chemistry to support production. The technologies we use include quantum chemical computation and molecular dynamics computation for simulating new materials. We introduced Matlantis in 2021, which allowed us to take a multifaceted approach to catalyst discovery.
Q. How does Matlantis differ from conventional simulations?
Tomokata Seki: Density functional theory (DFT) calculations are highly accurate and they can be applied for many types of materials including catalysts, but the bottleneck is that they are very time-consuming. With Matlantis, this becomes tens of thousands of times faster with almost the same accuracy. This had a considerable impact on me as a computational chemist. Our development division expects our computation speed and efficiency to keep pace with their operations. Matlantis paved the way all at once because the calculation speed is tens of thousands of times faster than the previous method.
Kento Sakai: In conventional catalyst development, we have mostly relied on experience, intuition, and literature to find materials and repeat experiments under gradually changing conditions. But what we wanted was to computationally predict reactions that we have never seen before, and we believe Matlantis will make that possible.
Takeda: To reach desired results in a reasonable frame of time, we previously had to screen candidate materials depending on priorities and compare their advantages and disadvantages. Matlantis frees us from that screening process, which allows us to look into the types of candidates that we used to avoid. We can now look into uncharted territories and make suggestions out of them.
Q. Has Matlantis changed your catalyst development process?
Ippei Furikado: We previously had to select what appeared to be the best candidates first because the calculations were too slow for the limited time that’s given to us. But now our mindset is to calculate everything that we can think of.
Sakai: The calculations on Matlantis are quick, but we cannot use the output as it is for other divisions. We built a system that predicts catalytic reactions using computational chemistry to cover what Matlantis doesn’t do, which is to combine simulation results in a way experimental scientists find useful (Figure 1).
Seki: In the past, when we couldn’t synthesize a desired material, it was difficult to pinpoint the cause, whether it was a problem with the experimental conditions or physical instability. It took a lot of time to figure that out. It would be a waste of time and effort to keep trying the conditions that had no chance. It’s like digging for treasure that has never been buried there. But if we use computers, we can speed up the development process by predicting the presence or absence of such treasure ahead of time.
Takeda: I have heard that our team successfully revised the experimental conditions to test catalyst stability because they had confidence in the calculation results.
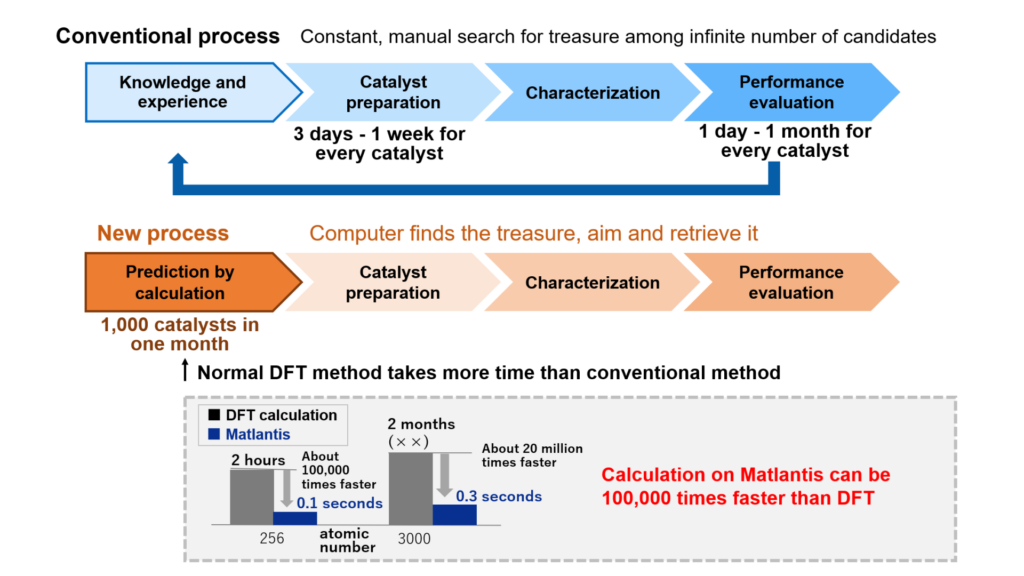
Q. Have there been any other changes?
Sakai: We need a certain size of calculation results for chemical studies. That used to take a lot of time but now I can quickly get enough results for that. It has given me some extra time to think about the issues that often arise, not about Matlantis itself, but about the unknowns in chemistry.
Seki: In the past, we often had to deal with development issues on a spot basis because they were time-consuming. But now that calculations are faster, I feel that the time lag between experimental scientists and computer scientists has decreased. We are now able to work together in each phase and process, and I have a much stronger sense that we are working together at a deeper level.
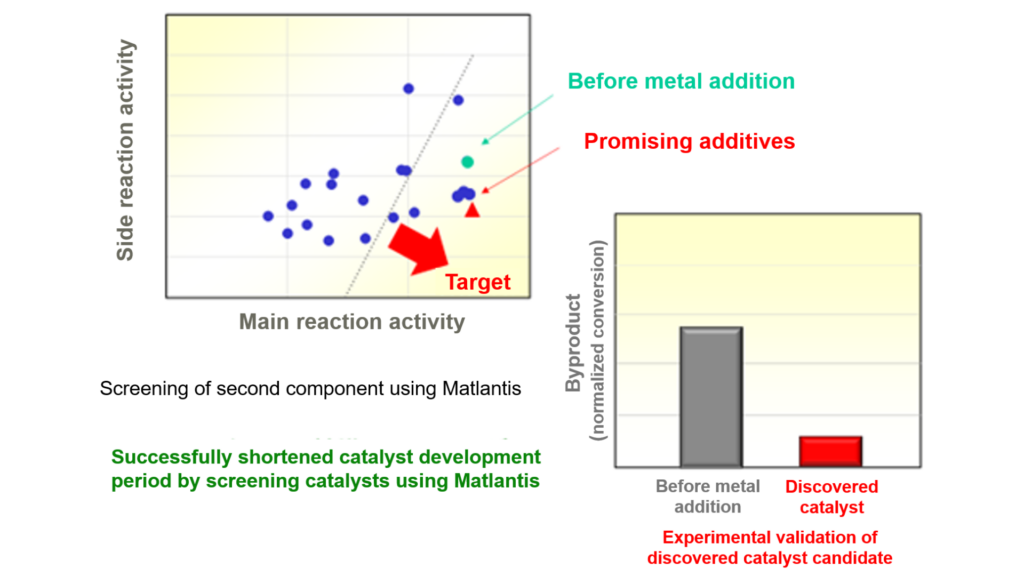
Q. What are your future goals with Matlantis?
Sakai: Kao’s bread and butter is detergents and cosmetics. Their development works the same way as our catalysts: They try many candidates to find what works the best. If computer simulation becomes the standard first step for our product development, I believe it will gain more support and understanding internally. Of course, we can’t apply the same technology for catalyst development as is, so additional development will be necessary.
Furikado: If experimental researchers can easily use Matlantis, I think it will be good news for the company as a whole. For example, it may help them predict and avoid potential experiment hazards.
Takeda: I believe that some of the features that Matlantis offers today could be applied to our main domain if they are put together in a right way. I hope to take advantage of Matlantis more to achieve big goals.
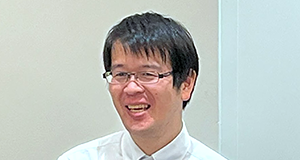
Ippei Furikado
Research and Development Division Analytical Science Laboratory
Group Leader
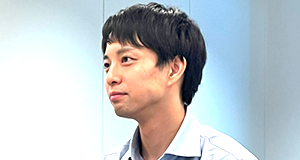
Kosuke Takeda
Theme Leader
In charge of introducing simulation methods, etc.
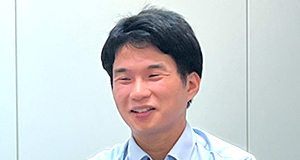
Kento Sakai
6th year with the company
In charge of predicting chemical reactions of catalysts
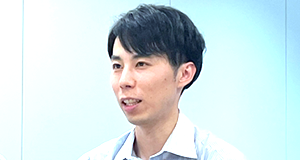
Tomotaka Seki
5th year with the company
In charge of stability prediction of inorganic materials
Kao Corporation
Founded in 1940, Kao operates a consumer products business as well as a chemical business that meets specific needs of the industry.
Address:1-14-10 Kayabacho, Nihonbashi, Chuo-ku, Tokyo
For more information, please visit the company website:https://www.kao.com/global/en/
Company names and product names appearing in this document are trademarks or registered trademarks of the respective companies. The information is current at the time of the interview.