Talk at CECAM Conference: Matlantis – Million years of research acceleration with universal neural network potential-based SaaS
Kosuke Nakago, Preferred Computational Chemistry, Inc. (PFCC), will give a talk titled “Matlantis – Million years of research acceleration with universal neural network potential-based SaaS” at Centre Européen de Calcul Atomique et Moléculaire (CECAM) conference.
Event Period
February 19 – 21, 2024
Location
Zuse Institute Berlin, Germany
Title
Matlantis – Million years of research acceleration with universal neural network potential-based SaaS
Abstract
Supercomputers play a vital role in addressing scientific challenges in atomistic simulations, including materials and drug discovery. Some research activities are important to achieve a future sustainable world, e.g., novel battery materials or hydrogen and synthetic fuel catalysts to solve energy problems. Due to the large number of potential candidates resulting from diverse combinations of atomic species, it becomes impossible to test all of them experimentally. As a result, high-throughput screening presents an appealing approach.
Traditionally, computational-heavy quantum chemistry calculations such as Density Functional Theory (DFT) and ab initio Molecular Dynamics (AIMD) are used, requiring supercomputers to handle large numbers of atoms. Breakthroughs have been brought from the deep learning field. Neural Network Potential (NNP) replaces DFT’s energy and force calculations with a surrogate model based on neural networks, significantly reducing computational costs.
Our research seeks to develop a versatile NNP model applicable to various systems with arbitrary combinations of atoms. We especially intensely focused on dataset collection and neural network architecture development.
One crucial aspect of our research involves extensive data collection to cover a wide range of simulation use cases. We have employed domain knowledge to curate a comprehensive dataset. We have covered various systems, including molecules, crystals, slabs, clusters, adsorption, and disordered systems. We also considered various states, e.g., optimized structures, vibration states, reaction states, etc., so that NNP can learn from various physical phenomena. This data collection effort has been enormous, with 33 million structures already calculated. It has required 1650 GPU years of computation in the last 4 years of research, and it is ongoing.
The other important aspect lies in developing a unique Graph Neural Network (GNN) architecture to achieve the universalness of NNP. Our architecture incorporates atom embedding to handle multiple atomic species while easily accommodating non-periodic and periodic systems. Our NNP’s rotational, translational, and permutation invariances ensure consistency with 3D coordinate input.
The research outcome has become an accessible SaaS called Matlantis. In 2023, 18 trillion atoms were successfully simulated with Matlantis in total. This corresponds to more than a million years of computation if done with DFT software on small computer clusters. In other words, massive pre-computation effort in dataset collection significantly accelerates the subsequent simulations executed by computational chemists worldwide, leveraging the trained universal NNP. In this regard, the universal NNP can be seen as a foundation model for atomistic simulations, analogous to the wide-ranging applications of foundation models like ChatGPT in various fields.
Matlantis is a SaaS for large-scale and high-throughput atomistic simulations that are traditionally done on supercomputers. Its inference efficiency is crucial. We also introduce our efforts to utilize AI accelerator MN-core to make computation even more efficient.
Lastly, we discuss the current limitations of our universal NNP research: handling macroscopic scale and microscopic electric phenomena.
In conclusion, we believe universal NNP can further accelerate computation-driven materials discovery, and overcome the limitations while enhancing the efficiency of atomistic simulations.
Speaker
Kosuke Nakago
Preferred Networks, Inc.
Kosuke Nakago is an Engineer at Preferred Networks, Inc. He received his master degree in theoretical physics from the University of Tokyo in 2014. He worked on deep learning research & development at Preferred Networks, Inc. and developed chainer-chemistry, A Library for Deep Learning in Biology and Chemistry. He is also supporting users to utilize Matlantis for their innovative material discovery at Preferred Computational Chemistry, Inc. His research interest includes deep learning as well as its application to materials science. He also participates data science competition and he is Kaggle competition master and notebooks grandmaster.
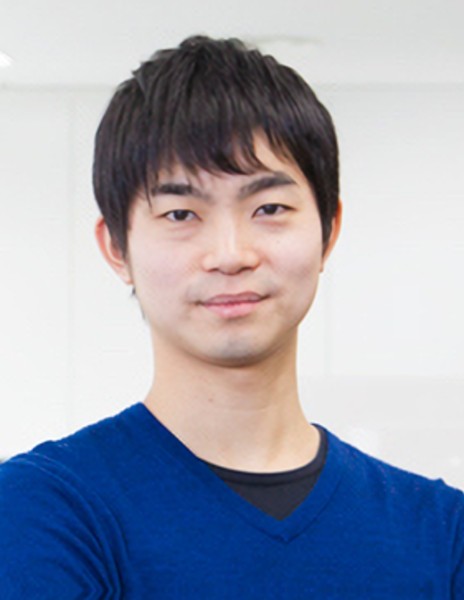
Rudy Coquet
Preferred Computational Chemistry, Inc.
Senior Manager at PFCC, he received his PhD in Chemistry from Cardiff University, UK in 2005 and his MBA from HEC Paris, France in 2013.
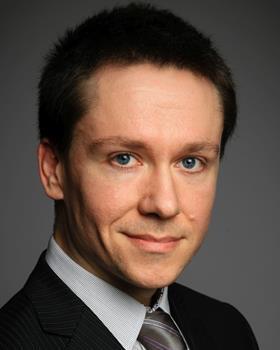