On-demand: History towards Universal Neural Network Potential for Material Discovery
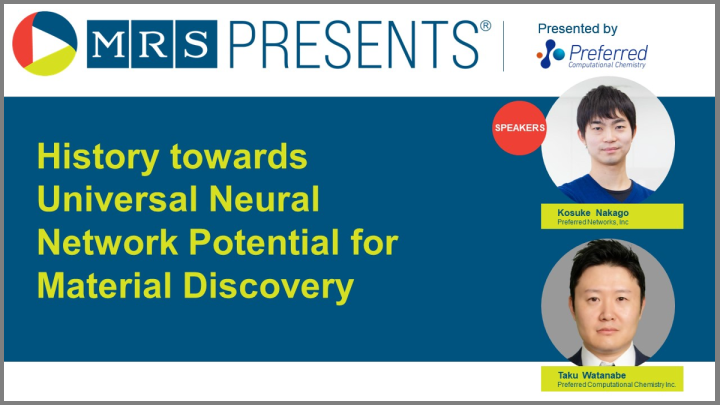
The on-demand webinar, “History toward Universal Neural Network Potential for Material Discovery,” is now available.
In collaboration with the Materials Research Society, Preferred Computational Chemistry hosted this webinar on October 4th, 2023.
Abstract
The rapid advancements of Artificial Intelligence technology have brought about revolutionary changes in materials discovery.
Neural Network Potential (NNP) describes molecular dynamics force field using a neural network, and many physical properties can be simulated using this single neural network. The webinar reviews the history of NNP research to understand how dataset & neural network architecture are improved.
We also describe the effort to develop a universal neural network and introduce the “PreFerred Potential (PFP)” implemented in Matlantis.
Who should attend
- Computational scientists who are facing limitations of their current calculation/simulation approach
- Researchers who are interested in learning game-changing atomistic simulation method
- Anyone who is interested in knowing about what’s happening at the forefront of materials informatics
Speakers
Preferred Networks, Inc.
Kosuke Nakago is an Engineer at Preferred Networks, Inc. He received his master degree in theoretical physics from the University of Tokyo in 2014. He worked on deep learning research & development at Preferred Networks, Inc. and developed chainer-chemistry, A Library for Deep Learning in Biology and Chemistry. He is also supporting users to utilize Matlantis for their innovative material discovery at Preferred Computational Chemistry, Inc. His research interest includes deep learning as well as its application to materials science. He also participates in data science competitions and he is Kaggle competition master and notebooks grandmaster.
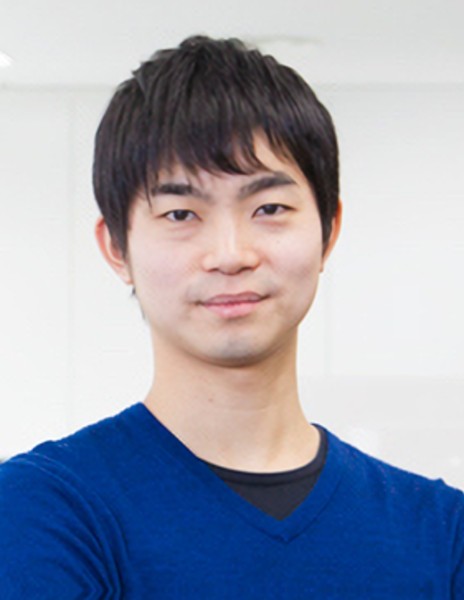
Preferred Computational Chemistry Inc.
Taku Watanabe, PhD. is a Lead Researcher at Preferred Computational Chemistry Inc. He earned his Ph.D. in Materials Science and Engineering from the University of Florida and did postdoctoral research in Chemical Engineering at Georgia Institute of Technology. In 2012, he joined Samsung R&D Institute Japan and dedicated his career for all-solid-state battery research for nearly eight years. His current research interest extends to battery materials, nanoporous solids, surface science, and the application of machine learning technology to computational chemistry in general.
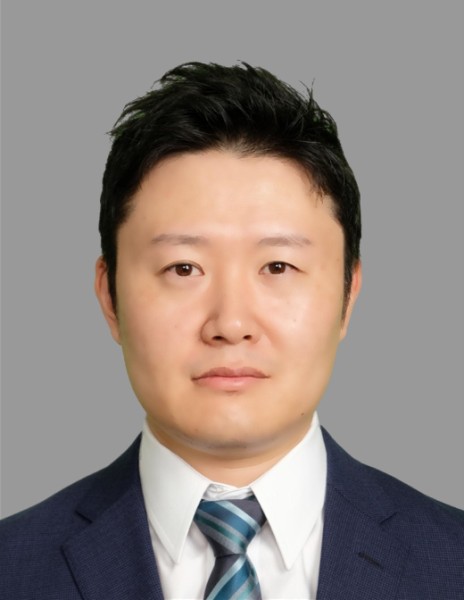