過去のお知らせ
展示会
Frankfurt am Main, Germany2024.6.10-14 (GER)
【ACHEMA 講演】Universal neural network potential for chemicals and materials discovery
ドイツを拠点とするプロセス産業の見本市、ACHEMAにて講演します。
ACHEMA公式website:https://www.achema.de/en/
開催期間
2024年6月10日~6月14日
場所
Frankfurt am Main, Germany
タイトル
Universal neural network potential for chemicals and materials discovery
概要
Atomic-scale simulations are making it possible to do more with complex chemistry than ever before. The rapid advancements of AI technology have brought about revolutionary changes in chemicals and materials discovery.
Such technology will be a vital component of a sustainable future, boosting efficiency for chemists at an individual level and allowing companies to handle increasingly large amounts of data while harnessing the numerous benefits of AI.
This presentation will first introduce a robust universal machine learning interatomic potential that covers much of the periodic table. More than one thousand GPU years were used to generate the ab initio training data guided by active learning. Diverse test simulations have shown this machine learning potential has outstanding performance, with energy error significantly less than the chemical accuracy (43 meV/atom) even for chemically very complex systems. This universal potential, which powers the Matlantis™ atomistic simulator, can run over 10,000 times faster than density functional theory (DFT) when dealing with several thousand atoms, and the latest release allows for more than 10,000 atoms of arbitrary combinations of 72 elements to be simulated together.
Focusing on use cases ranging from battery materials to lubricants and catalysts, the rest of the presentation will reveal how machine learning-based atomic scale simulations performed with Matlantis™ allow the study of realistic chemical systems, help rationalize experimental results, and foster the discovery of new chemicals. For example, it will be revealed how highly accurate and versatile machine learning models that link molecular structures to Radial Distribution Function (RDF) allowed the screening of tens of thousands of base oil compounds, resulting in the discovery of three compounds that could improve the processability of plastic working lubricants.
This presentation will be especially suitable for computational scientists who face the limitations of current simulation approaches and anyone interested in game-changing atomic scale simulations. No prior knowledge of the subject is required to take this first step towards large-scale chemicals and materials discovery, supported by state-of-the-art machine learning potentials.
発表者
Rudy Coquet
Preferred Computational Chemistry, Inc.
Senior Manager at PFCC, he received his PhD in Chemistry from Cardiff University, UK in 2005 and his MBA from HEC Paris, France in 2013.
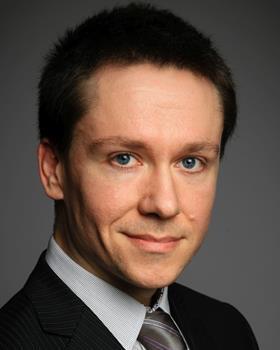
公開日:2024.05.07